Understanding SageMaker Endpoint Pricing for Businesses
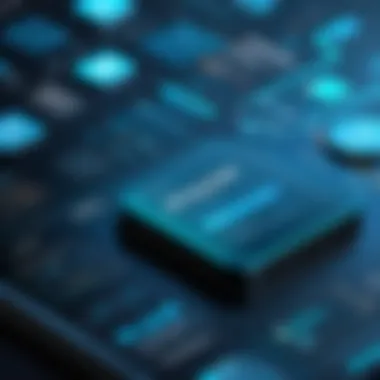
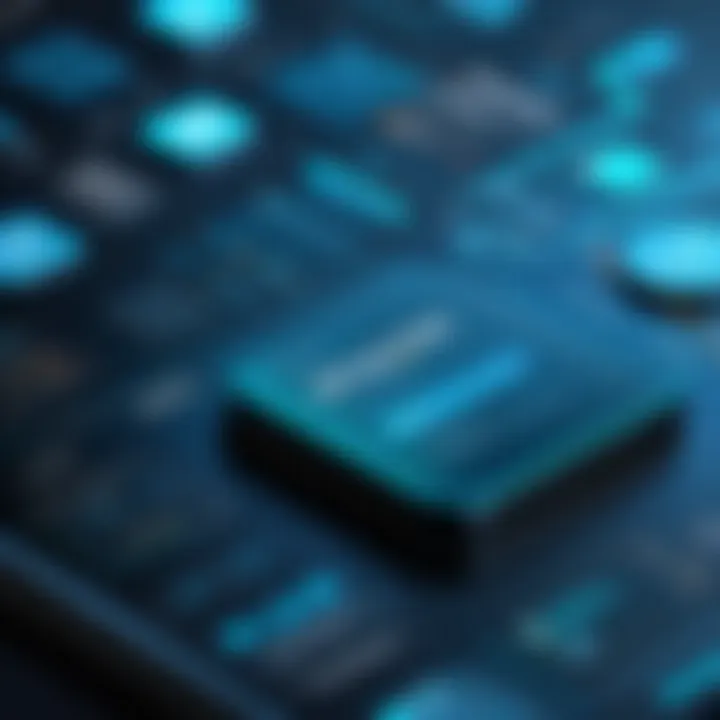
Intro
Amazon SageMaker is a comprehensive service designed to facilitate the building, training, and deployment of machine learning models. One of the key aspects of this service is the endpoints, which play a crucial role in making the trained models accessible for real-time predictions. Understanding the pricing structure of SageMaker endpoints is essential for businesses that seek to leverage machine learning without incurring excessive costs. This article aims to shed light on the various components of SageMaker endpoint pricing and provide insights on optimizing these expenses.
Exploring the pricing models and factors influencing costs can empower tech professionals and decision-makers to make informed choices. By managing resources wisely, businesses can maximize the value from their investment in this solution. In the following sections, we will delve deeper into the features, performance, and cost-saving strategies associated with SageMaker endpoints.
Prelude to SageMaker Endpoint Pricing
Overview of Amazon SageMaker
Amazon SageMaker is a comprehensive service that facilitates building, training, and deploying machine learning models at scale. It provides a suite of tools for every stage of the machine learning workflow. These tools empower developers and data scientists to automate the process of model training, thereby accelerating time to deployment. SageMaker eliminates much of the complexity traditionally associated with machine learning, allowing teams to focus on creating algorithms that deliver valuable insights.
Moreover, SageMaker endpoints allow for real-time predictions by serving the trained models. Each endpoint is a deployed instance capable of handling incoming requests for predictions. Understanding how these endpoints are priced can directly impact the budget dedicated to machine learning initiatives and influence decision-making in resource allocation.
Importance of Understanding Pricing
Understanding the pricing of SageMaker endpoints is paramount for businesses that intend to implement machine learning effectively. The flexibility and scalability offered by SageMaker come with financial implications that can vary significantly based on usage patterns, instance types, and the chosen pricing model.
Key considerations include:
- Budget Management: Having a clear grasp of the costs involved helps in effective budget planning. This insight can guide choices about the type of instances to use, how long to deploy endpoints, and when to switch pricing models.
- Cost Efficiency: Monitoring SageMaker costs can lead to actionable insights. Organizations can refine their usage, optimize resource allocation, and ultimately reduce unnecessary expenditures.
- Strategic Decision Making: Knowing endpoint pricing allows technical and managerial teams to make informed decisions when scaling operations or initiating new projects.
- Competitive Edge: In a market driven by data, controlling one’s costs can provide a crucial competitive advantage. Efficient use of resources often correlates with superior project outcomes in machine learning.
Components of SageMaker Endpoint Pricing
Understanding the components of Amazon SageMaker endpoint pricing is crucial for businesses that aim to leverage this service effectively. Each component incurs costs that can accumulate, affecting the overall budget. Therefore, gaining insight into these costs allows organizations to create a financial strategy that supports their machine learning initiatives.
Instance Cost
The instance cost represents the primary expenditure associated with the SageMaker endpoints. It refers to the amount charged for the runtime of compute instances. Different types of instances are available, and each comes with a distinct pricing structure. For example, GPU-based instances like have higher costs compared to CPU-based instances.
When selecting an instance type, organizations must consider the compute power required for their model. This power varies based on the algorithm and the volume of data being processed. It is also essential to remember that utilizing multiple instances simultaneously will linearly increase costs. Hence, judiciously choosing instance types can lead to significant savings.
Data Transfer Costs
Data transfer costs are another important aspect of SageMaker pricing. Charges apply when data is transferred between SageMaker and other AWS services, such as Amazon S3. This can also include data sent out to the internet. Understanding these costs is necessary to optimize data handling strategies within the overall architecture of machine learning projects.
For instance, keeping data in the same region reduces incurred charges effectively. Similarly, optimizing the volume of data sent to and from the endpoint can lead to cost reductions. It is prudent to create a clear data transfer plan before commencing your project for better expense management.
Storage Costs
The storage costs associated with SageMaker endpoints involve the expenses tied to storing models, datasets, and temporary files. These are generally linked to Amazon S3 where data can be stored and retrieved as needed. The pricing for storage typically depends on the total amount of data stored and the duration for which it is retained.
Organizations must assess their storage strategy to include lifecycle policies. Using such policies can help automate data archiving and, consequently, manage costs effectively. Additionally, cleaning up unneeded data can eliminate unnecessary charges.
To summarize, gaining a comprehensive understanding of the components of SageMaker endpoint pricing is vital for effective budgeting and resource allocation in machine learning projects. By paying attention to instance costs, data transfer costs, and storage costs, businesses can make informed decisions.
Pricing Models for SageMaker Endpoints
Understanding pricing models for SageMaker endpoints is crucial. It allows businesses to align their machine learning expenditures with their specific needs and utilization habits. Each pricing model offers distinct advantages, catering to different usage patterns and operational strategies. By comprehending these models, organizations can make informed decisions that optimize costs while maximizing the efficiency of their machine learning deployments.
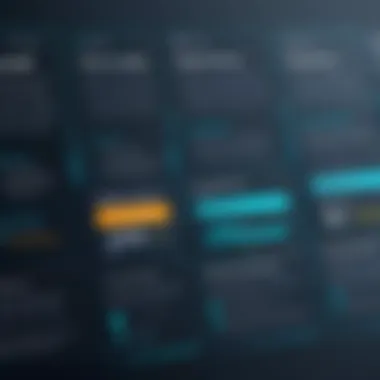
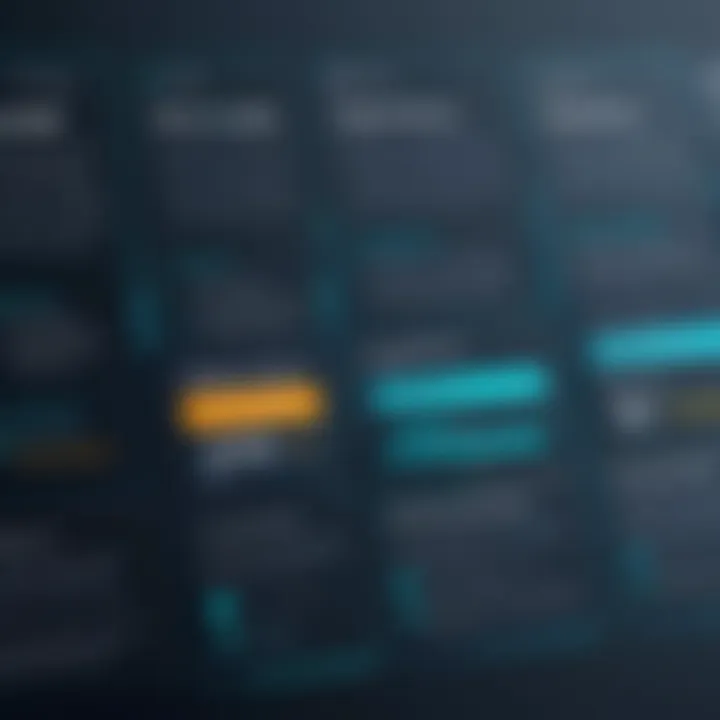
On-Demand Pricing
This pricing model provides flexibility. Users pay for SageMaker instances on an hourly basis, meaning that costs are incurred only when the instances are running. This can be beneficial for projects with unpredictable workloads or development phases where instances are needed intermittently.
Benefits of on-demand pricing include:
- No long-term commitment.
- The ability to scale up or down quickly.
- Ideal for short-term projects.
However, organizations must carefully manage usage. Without oversight, on-demand pricing can lead to unexpectedly high costs if instances are left running for extended periods. This model is most suitable for those who need maximum flexibility without the burden of commitment.
Spot Pricing
Spot pricing is potentially the most cost-effective option for users who can tolerate interruptions. Amazon offers unused capacity at reduced rates, allowing users to bid on instances. While the savings can be significant, it is essential to understand the trade-offs.
Key points about spot pricing include:
- Cost savings: Discounts compared to on-demand instances.
- Unpredictability: Instances can be terminated with little notice, making it unsuitable for critical applications.
- Bidding process: Users often bid low and may need to adjust their bids according to available capacity.
This model is ideal for batch jobs, data processing, or any workload where interruptions are manageable. Being aware of the nature of your tasks is key to effectively using this pricing model.
Reserved Instances
Reserved instances offer a different approach, targeting users who have predictable workloads. By committing to a specific instance type for a one- or three-year term, customers can secure significant discounts compared to on-demand pricing.
The advantages of reserved instances include:
- Cost efficiency: Upfront payments lead to lower hourly rates over time.
- Predictability: Well-suited for applications with consistent resource needs.
- Capacity reservation: Confidence that specific resources are available when needed.
While this model can save costs in the long run, it does involve a commitment that may not be suitable for all users. Organizations should evaluate their future needs carefully to determine if and when to utilize reserved instances.
Overall, understanding these pricing models enables organizations to align their operational requirements with cost management strategies when utilizing SageMaker endpoints. The choice among these models significantly impacts the overall financial efficiency of machine learning projects.
Factors Influencing SageMaker Endpoint Costs
Understanding the factors influencing the costs associated with Amazon SageMaker endpoints is crucial for any organization utilizing this machine learning service. By grasping these underlying elements, businesses can make informed decisions that optimize their spending while harnessing the power of machine learning. Each factor interplays with others and can lead to varying implications on budget management.
Instance Type Selection
Selecting the appropriate instance type is a decisive factor in determining the costs for using SageMaker endpoints. Amazon offers a variety of instance types tailored for different computational needs, each with its own cost structure. For instance, the compute-optimized instances are typically more expensive than general-purpose ones. Furthermore, larger instances not only carry a higher hourly rate but can also lead to increased efficiency for complex models, offsetting costs by reducing processing time. It is essential to analyze workload requirements carefully before committing to a specific instance type. Factors such as the expected traffic, model complexity, and concurrent users should be integral to this decision.
Endpoint Usage Patterns
The patterns in which endpoints are utilized directly affect the associated costs. Understanding how often and when your endpoints are used can lead to significant savings. For example, endpoints that are turned on 24/7 will accumulate costs differently compared to those that are activated only during peak hours or specific intervals. This invites consideration of cost-effective practices like scheduling endpoint availability to align with peak usage times. Additionally, organizations can take advantage of SageMaker’s automatic scaling options, which can adjust capacity based on real-time demand, further optimizing cost effectiveness over time.
Model Complexity
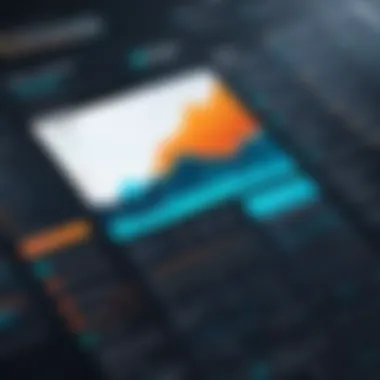
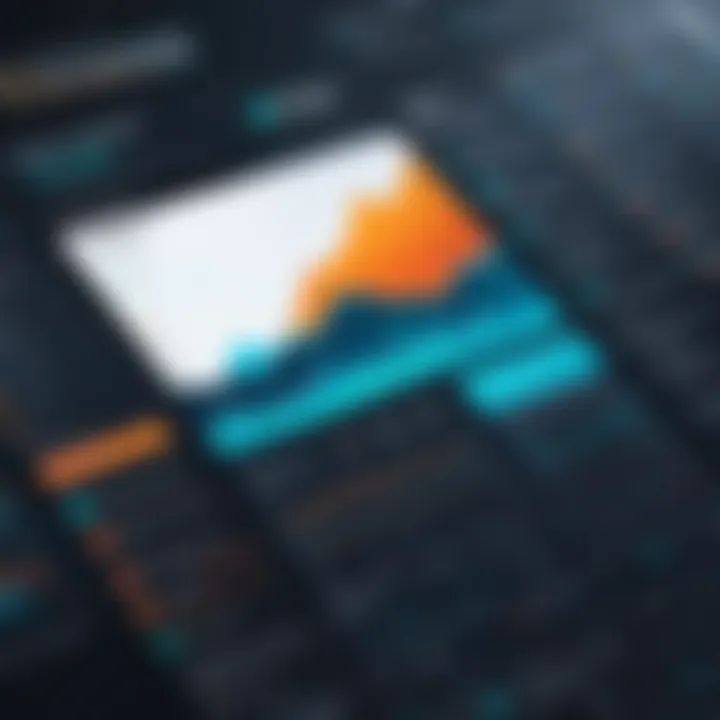
The complexity of the models deployed on SageMaker endpoints also has a noteworthy impact on overall costs. More complex models often demand higher computational resources, which can escalate costs quickly. It is vital to evaluate whether the gains from a sophisticated model substantiate its operational expenses. Simplifying models or implementing model optimization techniques can mitigate costs while still ensuring adequate performance. Examining pre-trained models or leveraging transfer learning are viable approaches to consider in achieving cost efficiencies.
"A thorough understanding of these factors aids in the formulation of a detailed cost management strategy, leading organizations to leverage SageMaker more effectively."
Cost Management Strategies
Effective cost management strategies are critical to optimizing expenses associated with Amazon SageMaker endpoints. These strategies go beyond traditional accounting methods and delve into the operational aspects that contribute to overall spending. For tech professionals and IT decision-makers, understanding how to manage costs can lead to significant savings while maximizing resource utilization.
Having a well-defined strategy in place enables organizations to monitor expenses in real-time and make informed decisions. This is important because the machine learning landscape is ever-evolving, and costs can escalate quickly without proper oversight. By focusing on specific elements such as monitoring usage, scaling instances optimally, and employing cost management tools, businesses can ensure they do not overspend on services that can be adjusted or optimized.
Monitoring Utilization
Monitoring utilization is the first step in effective cost management. This involves keeping track of how resources are being used in real-time. Organizations should establish metrics to evaluate usage patterns. Are instances consistently underutilized? Is there a spike in usage during specific times?
By gaining insights from this data, companies can make data-driven decisions. They can choose to downsize underused instances or consolidate workloads to reduce costs. Tools such as Amazon CloudWatch can provide valuable insights into resource utilization, enabling teams to understand their consumption patterns deeply.
Optimal Instance Scaling
Optimal instance scaling refers to adjusting the size and number of instances based on current needs. Businesses often face fluctuating workloads. For instance, during peak periods, they may require more resources, while during off-peak times, excessive resources can lead to unnecessary expenses.
To achieve optimal scaling, organizations should adopt auto-scaling policies. This allows SageMaker to automatically adjust the number of instances based on current workloads. Such strategies ensure that the service operates efficiently while minimizing costs.
Employing Cost Management Tools
Employing cost management tools is another pivotal strategy in managing SageMaker endpoint expenses. Tools like AWS Budgets or AWS Cost Explorer provide insights into spending trends and forecasts. By setting budget alerts, organizations can receive notifications when costs approach defined thresholds.
Additionally, integrating third-party solutions that offer advanced analytics can further enhance understanding of spending patterns. These tools can identify areas of unnecessary expenditure, thus enabling businesses to act quickly.
In summary, adopting a comprehensive approach to cost management strategies can lead to significant savings in Amazon SageMaker endpoint expenses. By monitoring utilization, scaling instances optimally, and utilizing cost management tools, organizations can strike a balance between operational efficiency and cost-effectiveness.
Comparison with Other Machine Learning Services
Comparing Amazon SageMaker Endpoint pricing with other major machine learning services is crucial for businesses seeking value and optimization. Understanding how these platforms measure up against SageMaker can influence critical decision-making regarding resource allocation, cost management, and efficiency. Given the investment often required for machine learning solutions, making informed choices is exceptionally important.
There are several elements to consider in this comparison:
- Cost structures: Examining the different pricing models across services helps to determine the most economical choice for specific workloads.
- Feature sets: Evaluating included functionalities and available resources can provide insights into potential costs that might arise when using alternative services.
- Scalability and flexibility: Understanding how each platform scales with demand can affect long-term expenses. Different services may offer varying levels of control over scaling operations.
By thoroughly comparing these elements, tech professionals can make strategic decisions aligned with their financial and operational goals.
Cost Comparison with Google AI Platform
Google AI Platform offers a competitive alternative to SageMaker. When comparing costs, several aspects should be considered. Google AI Platform charges for compute resources utilized during model training and inference. This pricing can be influenced by instance types selected and the duration of use. A notable element is the availability of preemptible VM instances, which can significantly reduce costs but come with caveats regarding availability.
For businesses that utilize heavy workloads, this feature allows for possible cost-saving, but it requires careful planning. An additional cost factor is data storage and transfer, dependent on the volume of data processed, making real comparisons essential. Overall, while Google's pricing may be favorable in certain scenarios, the actual cost-effectiveness can vary widely based on the project’s nature and specifics.
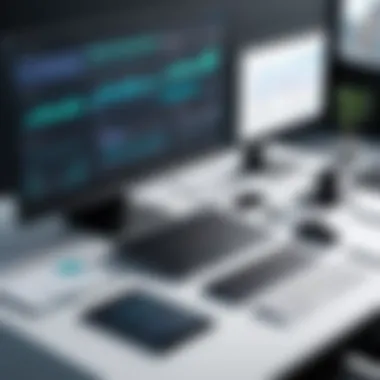
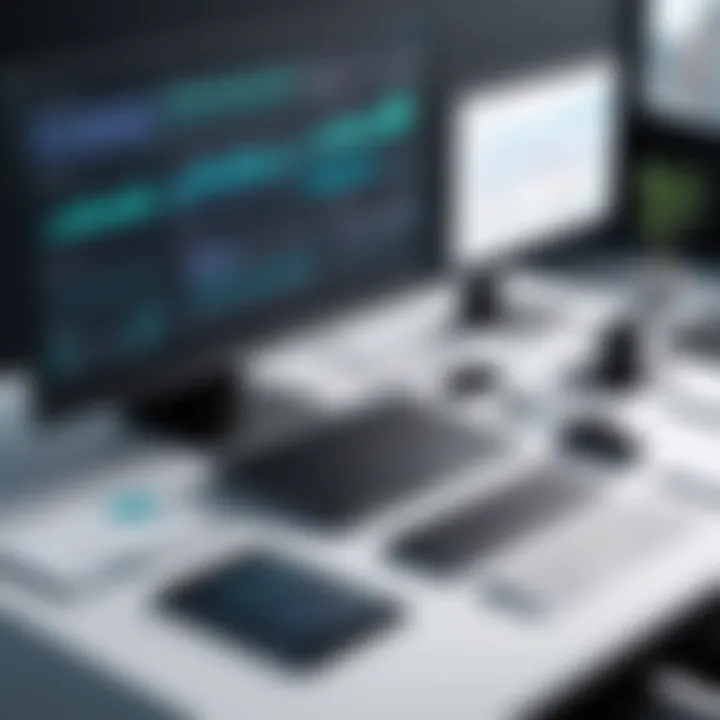
Cost Comparison with Microsoft Azure
Microsoft Azure ML presents another viable competitor in the realm of machine learning services. Azure ML pricing is structured around compute resources, with options for pay-as-you-go or reserved instances. Lazy loading capabilities and automated machine learning can lead to efficient resource usage, impacting overall expense positively.
One of the key points is the option for low-priority VMs, similar to Google’s preemptible VMs, which can be used to achieve lower operational costs during non-critical workloads. Users also have an option to integrate Azure Machine Learning with other Azure services, which may lead to synergistic savings depending on existing infrastructure. Users should take a closer look at the total cost impact of the services they are planning to implement, factoring in possible hidden costs.
Real-World Case Studies
Real-world case studies serve as a vital lens through which we can examine the practical implications of SageMaker endpoint pricing. They provide concrete examples of how different organizations leverage Amazon SageMaker to optimize their machine learning activities while managing costs effectively. Understanding these cases enables us to extract valuable insights that can be applied across various sectors. By analyzing how these businesses have approached pricing, we can identify common strategies and challenges that arise.
Enterprise Implementation
In large enterprises, implementing Amazon SageMaker often involves substantial investment, driven by both infrastructure and human resources. A notable example is a major retail chain that deployed SageMaker to enhance their inventory forecasting models. By utilizing on-demand pricing for their endpoints, the company was able to keep costs in check while benefiting from the flexibility to scale resources when needed.
The enterprise approach revealed several considerations:
- Scalability Needs: The retail chain adopted a usage pattern that varied with seasonal demand. Their strategy included adjusting the number of instances during peak shopping times, reducing expenses during slower periods.
- Budgeting Complexity: The finance department leveraged tools like AWS Cost Explorer to track expenditures related to the endpoints. This helped them forecast future costs based on historical usage.
- Collaboration Across Teams: Involving multiple departments, from IT to marketing, helped ensure that the deployment aligned well with business goals while being mindful of costs.
These factors contributed to an overall positive return on investment, demonstrating that with careful planning and execution, enterprises can exploit SageMaker’s capabilities effectively.
Startups and Cost Efficiency
Startups, on the other hand, often have tighter budgets and must prioritize cost efficiency. Many look to SageMaker for its potential to streamline operations without large upfront expenses. A prominent startup in the tech industry implemented SageMaker for predictive analytics. Their focus was on maximizing value while keeping costs low.
Key elements of their approach included:
- Spot Pricing Utilization: This startup took advantage of spot instances for their non-urgent workloads, significantly cutting down on costs compared to traditional on-demand prices. Spot instances allowed them to use AWS resources flexibly without compromising on performance.
- Experimentation and Learning: By integrating SageMaker’s notebook instances with an iterative development process, they managed to develop several models at a fraction of the traditional costs. They fine-tuned algorithms based on real-time feedback, enabling quicker adaptations to user needs.
- Partnerships and Resources: Many startups leveraged the AWS Activate program, which provided credits and technical support. This support reduced initial costs and allowed them to focus resources on development.
Through these case studies, it becomes evident how both large enterprises and startups can strategically leverage Amazon SageMaker. The diversity of usage samples also highlights the importance of adapting strategies to fit specific organizational needs and objectives.
Ending and Future Trends
In the context of Amazon SageMaker Endpoint Pricing, the conclusion serves as a pivotal juncture for those exploring the intricate landscape of costs involved in machine learning deployment. Understanding pricing is not merely an academic exercise; it has real-world implications for budgetary planning and operational efficiency within organizations. The insights gained from this analysis can inform strategic decision-making, enabling businesses to optimize their resource allocation.
This section emphasizes the ongoing evolution of pricing structures within the field of cloud computing. Technology and market trends fluctuate rapidly, which means that staying abreast of changes is crucial for any tech professional. Understanding future pricing trends can facilitate better risk management and investment strategies.
"Making informed decisions based on current pricing models and anticipating future changes is vital for maximizing the ROI in cloud machine learning services."
Summary of Key Points
The key points discussed throughout the article highlight several critical aspects of SageMaker endpoint pricing:
- Pricing Components: The costs associated with instance usage, data transfer, and storage directly impact the overall expenditure.
- Pricing Models: Options such as on-demand, spot pricing, and reserved instances provide flexibility, allowing businesses to choose models that best suit their needs.
- Cost Influencers: Factors that affect pricing, including instance types, usage patterns, and model complexity, can lead to significant differences in overall costs.
- Cost Management Strategies: Effective monitoring and optimization practices can markedly improve cost efficiency.
- Comparative Analysis: Comparing SageMaker with alternative platforms like Google AI and Microsoft Azure helps stakeholders understand relative cost frameworks.
Potential Developments in Pricing
The future of SageMaker endpoint pricing is likely to reflect broader industry trends in both artificial intelligence and cloud services. Several potential developments may shape pricing strategies moving forward:
- Dynamic Pricing Models: As businesses become more data-driven, we could see the emergence of more sophisticated, real-time pricing models that adjust according to demand and usage patterns.
- Increased Granularity in Pricing: Future iterations may offer more nuanced pricing tiers based on specific features utilized or customer behavior, providing greater flexibility in budgeting.
- Integration of AI and Machine Learning: Enhanced predictive algorithms could lead to improved pricing models, allowing companies to forecast costs more accurately based on usage patterns.
- Competitive Pressures: As competition among cloud providers intensifies, effective pricing strategies will be paramount for retaining customers and market share.
- Regulatory Developments: Changes in global data policy and compliance requirements could also influence pricing structures as companies adapt to new legal frameworks.
Overall, staying informed about these future trends will be essential for anyone engaged in deploying machine learning services on Amazon SageMaker.