Discovering Free Data Science Courses on Coursera
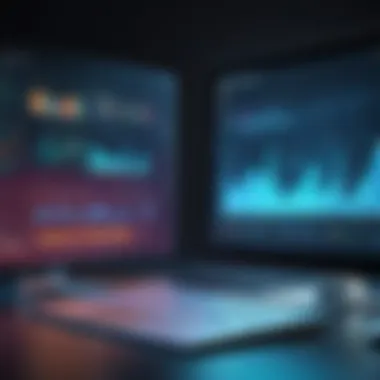
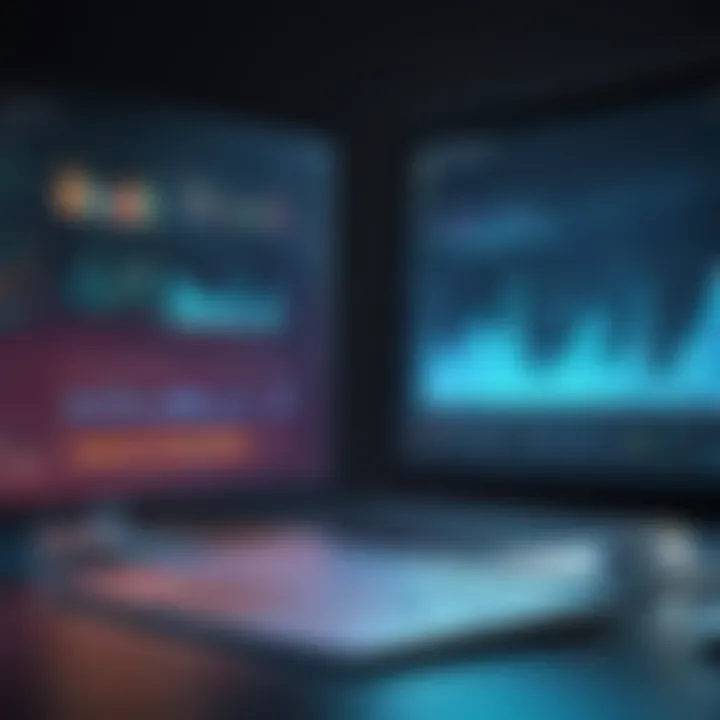
Intro
In today’s data-driven world, understanding data science isn't just a luxury—it’s a necessity. For anyone from software developers to budding analysts, knowledge in this field can dramatically enhance career prospects. However, the costs of education can be a stumbling block. Thankfully, Coursera offers a treasure trove of free data science courses for eager learners.
People often wonder how to navigate this vast offering. Knowing which courses suit one’s knowledge level, goals, or interests becomes crucial. This guide aims to spotlight the gems hidden in Coursera’s catalog, providing clear insights into course structures, key topics, and practical applications. With a focus on unique selling points and evaluations of performance, this article facilitates informed decision-making around engaging with data science education.
Key Features
Overview of Features
Coursera's free data science courses come neatly packed with various features designed to foster a rich learning environment. Here’s a closer look at some highlights:
- Diverse Course Offerings: Whether your interest lies in machine learning, statistics, or programming, there’s something for everybody.
- Flexible Learning Structure: Participants can learn at their own pace—no deadlines to stress over means more focus on genuine understanding.
- Hands-On Projects: Many courses include real-world projects that allow learners to apply their knowledge in practical ways. This not only solidifies learning but also builds a robust portfolio, proving beneficial for job applications.
- Skill Assessments: Quizzes and tests sprinkled throughout the courses help gauge progress, ensuring that concepts are understood before moving on.
Unique Selling Points
What makes these courses stand out in the crowded online learning landscape? Here are some unique aspects of Coursera's offerings:
- Collaborations with Renowned Institutions: Many courses are crafted by respected universities and institutions globally, such as Stanford and IBM. This adds credibility to the qualifications gained.
- Succinct and Relevant Content: Courses cover a wide spectrum of current topics and tools in data science, ensuring that learners stay relevant in an ever-evolving field.
- Accessible Learning Materials: Most resources, lectures, and readings are easily accessible and designed to cater to varying levels of experience. The materials often include video lectures, reading materials, and interactive elements.
"The right course can open doors. With so many available for free, now is the time to dive into data science!"
Performance Evaluation
Speed and Responsiveness
The platform itself is user-friendly. Course navigation is swift, with minimal loading times, allowing users to transition smoothly between lectures and assignments. This matter of speed can make a significant difference, especially for those who juggle multiple responsibilities.
Resource Usage
Coursera has optimized its courses to require a reasonable internet bandwidth. This means that students can partake even on less robust connections. The app also supports offline learning, letting participants download materials to review at their convenience. This flexibility can be pivotal for those on a tight schedule.
Prelims to Data Science Education
Data science has woven itself into the fabric of modern society, influencing decisions in businesses, healthcare, education, and countless other fields. This section sets the stage for understanding data science education and its implications in today’s world. It’s crucial to grasp the underpinnings of this knowledge domain, given its increasing relevance.
Understanding Data Science
At its core, data science is an interdisciplinary blend of statistics, mathematics, programming, and domain expertise. It involves collecting, processing, analyzing, and interpreting large datasets to uncover patterns and support decision-making. One might think of it as a detective work that leverages technology and analytics. The notable aspect here is that data isn’t just figures in spreadsheets; it tells stories, shaping narratives based on real-world complexities. The growth in available data has made mastering these skills paramount—not just for data scientists but for anyone working in data-driven industries.
The foundational competencies in data science include:
- Statistical Knowledge: Understanding the principles of statistics aids in grasping data distributions and variable relationships.
- Programming Skills: Familiarity with programming languages like Python or R is vital for data manipulation and analysis.
- Data Visualization: Tools such as Tableau or Matplotlib help in creating intuitive visuals that make complex data understandable.
- Domain Knowledge: Knowing the context in which you operate allows better insights from the data.
Especially for professionals already grounded in tech fields, picking up data science can feel less like starting anew and more like refining existing skills. The integration of statistical analysis with programming can complement their current skill set, making them increasingly valuable in their respective workplaces.
Importance of Online Learning
With the evolution of technology and the internet, learning has taken a new dimension. The accessibility of knowledge has surged, and data science is at the forefront of this shift. Online platforms like Coursera provide exhaustive resources for those eager to learn but unable to commit to traditional education pathways.
The advantages of online learning in data science are numerous:
- Flexibility: Individuals can learn at their own pace, fitting education into an already tight schedule.
- Variety of Courses: There’s a multitude of free resources. From beginner to advanced topics, learners can select what suits their needs.
- Diverse Community: Online platforms connect learners globally, allowing for cooperative learning and sharing of ideas.
- Cost-Effectiveness: Many online courses are free, creating opportunities for those who might lack financial means.
"The accessibility of online courses democratizes education, providing training that was once only available to those enrolled in traditional institutions."
By breaking geographical barriers, online learning opens up paths for young professionals, students, and even seasoned experts to upskill. The result is a workforce that is not only well-trained but also equipped to handle the demands of a data-driven economy. In essence, as one immerses in data science via these platforms, they become part of a larger movement towards continual learning in today’s fast-paced world.
Overview of Coursera
In this age of digital information, understanding what platforms like Coursera offer is crucial. Coursera is more than just an online learning platform; it's a global hub for education that brings together prestigious universities and renowned companies. By providing a vast array of courses, especially in data science, Coursera plays a pivotal role in shaping educational pathways for learners everywhere. That can't be overlooked.
What is Coursera?
At its core, Coursera is a web-based platform that partners with leading educational institutions to offer courses without geographical limitations. Founded in 2012 by Stanford professors Andrew Ng and Daphne Koller, Coursera serves as a bridge between traditional education and online learning. It has transformed the learning experience by allowing individuals to engage with high-quality educational material at their own pace.
Some defining features of Coursera include:
- Wide Range of Subjects: From computer science to humanities, the subjects available cater to various interests and career goals.
- Collaborative Learning: It encourages peer-to-peer interaction, enabling students to collaborate and share insights.
- Accessible Learning: Not bound by time zones or rigid schedules, learners can pursue courses on their own terms, which is a significant advantage.
Coursera's Role in Education
The role of Coursera extends well beyond merely providing content. It’s a platform that democratizes education, making it available to anyone with an internet connection. This has profound implications, especially for those in remote areas or those who cannot attend conventional institutions due to financial or personal constraints.
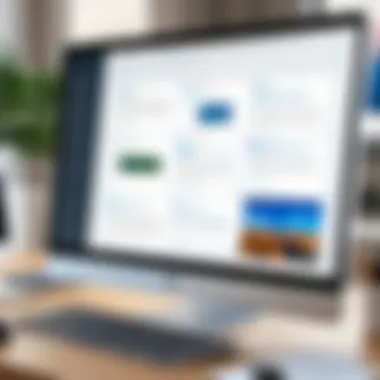
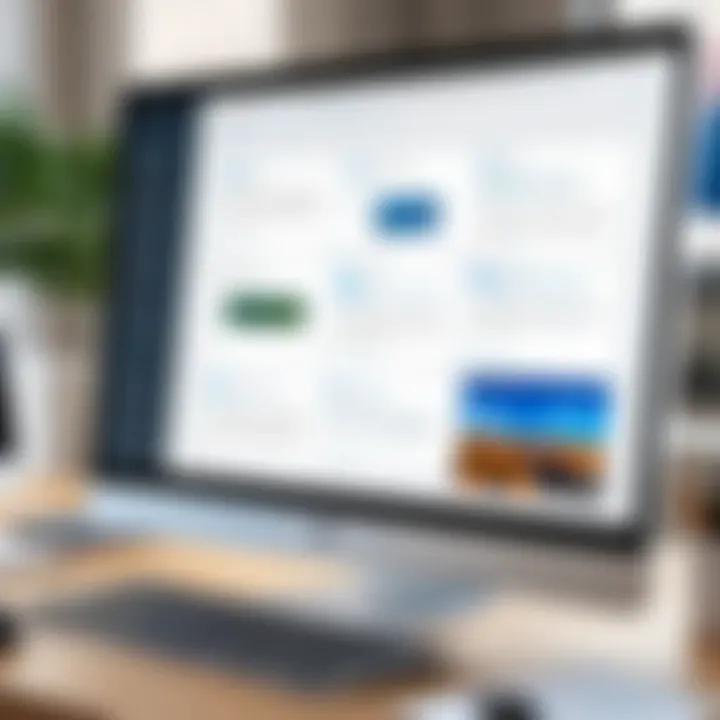
Coursera offers numerous free courses, including those in data science, making learning accessible for everyone. By completing these courses, learners can not only gain knowledge but also earn certificates that enhance their resumes.
"Coursera is not just about learning; it's about opening doors to opportunities that may have seemed impossible before."
The platform also adapts to the evolving educational landscape by constantly updating its offerings. For professionals, this is invaluable, as it allows for continual upskilling, a necessity in fields like data science that are in constant flux.
Overall, understanding what Coursera brings to the table is essential for anyone considering diving into data science. Whether it’s initial forays into the subject or more advanced courses, the insights gained will equip learners with tools for your future endeavors.
Free Data Science Courses on Coursera
The realm of data science is expansive, and courses offered on Coursera serve as gateways to enter this bustling field. Free data science courses in particular are a lifeline for many aspiring data professionals, allowing them to build foundational knowledge without the burden of financial constraints. It’s an avenue where anyone, regardless of their background, can start their journey in data science. These free offerings break down barriers and democratize access to quality education.
List of Free Courses Available
Coursera hosts a wide array of free data science courses, catering to various skillsets and interests. Here's a brief rundown of some notable ones:
- Data Science Specialization by Johns Hopkins University: This program is structured as a series of courses that guide learners through the entire data science pipeline. It covers R programming, data manipulation, and machine learning techniques.
- Introduction to Data Science in Python by University of Michigan: This is an excellent course for those who are keen on learning the basics of data science using Python. It focuses on data analysis and visualization.
- Machine Learning by Stanford University: Led by renowned professor Andrew Ng, this course is groundbreaking. It covers fundamental machine learning algorithms and their real-world application.
- Data Visualization with Python by IBM: This course targets those who wish to express their data findings visually. It provides insights into various visualization libraries available in Python.
- Applied Data Science with Python Specialization by University of Michigan: Delving deeper, this specialization teaches learners how to apply statistical techniques using Python, which is invaluable in today’s data-driven roles.
Each of these courses has been thoughtfully crafted to ensure learners achieve not only theoretical understanding but also practical insights. Consider checking out the Coursera website to see the updated list, as they continuously add new courses.
How to Access Free Courses
Getting into these free courses is quite straightforward, but there are a few steps to keep in mind:
- Create a Coursera Account: Navigate to Coursera's homepage and sign up. This requires just an email and a password. You might also use your existing Google or Facebook account for quicker access.
- Search for Courses: Utilize the search bar. Simply type in "Data Science" to filter relevant free courses available on the platform.
- Enroll in Courses: After selecting a course, hit the "Enroll for Free" button. In most cases, you can join the course without any charge. Some courses offer a “paid certificate” option, but you can opt-out of that if your focus is solely on learning.
- Engage with Course Material: Once enrolled, start accessing the materials at your own pace. It's advisable to structure your time wisely, dedicating regular hours each week to cover modules, complete assignments, and participate in discussions.
- Join Discussion Forums: Engaging with peers and instructors via community forums can enhance your learning experience. You might find solutions to queries and even network with others in the same learning trajectory.
This streamlined access means that anyone interested can jump in and begin enriching their skillset right away, with just a click of a button.
Course Contents and Structure
Understanding the course contents and structure of data science classes on platforms like Coursera is critical to discerning which classes fit best with one's learning goals. Often seen as the backbone of any educational journey, the course material can make the difference between acquiring a superficial knowledge about data science and gaining a well-rounded understanding of complex concepts.
Importance of Course Structure
A well-structured course provides learners with a roadmap, guiding them through topics in a logical and coherent manner. For many, stepping into data science can be overwhelming. Without a clear structure, learners may find it daunting to navigate through vast amounts of information. Additionally, comprehensively designed courses often include quizzes, assignments, and interactive elements that reinforce the concepts covered.
There are several key elements worth considering when evaluating the course contents on Coursera:
- Relevance: Does the course cover the topics most pertinent to the current industry demands?
- Diversity of Topics: Are foundational topics such as statistics, programming, and machine learning included?
- Practical Aspects: Is there an emphasis on practical applications and projects?
Typical Topics in Data Science Courses
Data science is a multifaceted field, and free courses on Coursera usually encompass a broad range of topics. Let's delve into some typical subjects that these courses often cover:
- Introduction to Data Science: This usually serves as an overview of what data science is, encompassing its significance and applications in various industries.
- Statistics and Probability: Foundational concepts in statistics are critical in data analysis. Courses cover descriptive statistics, inferential statistics, and various probabilistic models.
- Programming with Python or R: Being adept in programming languages like Python or R is indispensable. Most courses offer hands-on exercises to build practical coding skills.
- Machine Learning: A segment dedicated to machine learning algorithms is common. Learners typically explore supervised and unsupervised learning techniques, including regression, classification, and clustering.
- Data Visualization: This topic covers tools and techniques used to visually interpret and present data. Familiarity with platforms like Tableau or libraries such as Matplotlib is often included.
- Big Data Technologies: Some courses touch on modern technologies for handling large data sets, such as Hadoop and Spark.
These topics not only equip learners with essential skills but also make them more attractive candidates in the job market.
Course Delivery Format
Delivery format also drastically affects how content is absorbed. Coursera employs various formats to cater to different learning styles:
- Video Lectures: These are often pre-recorded sessions that one can watch at their own pace. They typically integrate slides and live coding demonstrations.
- Interactive Quizzes: Designed to reinforce understanding, quizzes ensure learners are keeping pace with the material and grasping essential concepts.
- Capstone Projects: Many courses end with a capstone project, allowing students to apply everything they've learned in a real-world scenario. This element can be crucial in consolidating knowledge.
- Forums for Discussion: A platform for interaction amongst peers enhances the learning experience. Students can pose questions, share insights, and collaborate with others, enriching their understanding through social learning.
Learning Outcomes
Understanding the learning outcomes of free data science courses on Coursera is key for anyone looking to expand their skills in this dynamic field. These outcomes reflect what learners can expect to achieve by the conclusion of each course, whether they are a seasoned IT professional or a newcomer to the data science realm. Getting a clear picture of these benefits helps students select the offerings that align with their goals and aspirations.
Skills Acquired from Free Courses
Diving into free data science courses on Coursera can equip learners with a wide array of valuable skills. These courses typically cover essential topics like statistics, machine learning, and data visualization. For instance, a course focusing on Python for data analysis not only teaches the programming language but also delves into libraries such as Pandas and Matplotlib.
Here are some specific skills that participants can gain:
- Statistical Analysis: Understand how to interpret data and make informed decisions based on statistical insights.
- Programming Skills: Learn programming languages like Python or R, which are crucial for handling data.
- Machine Learning Techniques: Get acquainted with algorithms and methodologies for predictive modeling and data mining.
- Data Visualization: Acquire the know-how to present insights using visuals, making complex data more accessible.
- Problem Solving Abilities: Develop analytical thinking to tackle real-world data challenges effectively.
These skills not only enhance one's knowledge but are also highly sought after in today’s job market.
Practical Applications of Learned Skills
The knowledge and skills gained through free data science courses have practical applications that extend far beyond the classroom. Each skill cultivates a unique capability that can be applied in various scenarios. For example, expertise in data visualization can help present business insights effectively during meetings, leading to data-driven decision making.
Here are a few real-world applications:
- Business Analytics: Companies use data science skills to interpret trends and drive strategy.
- Healthcare Improvements: Data science can transform patient care through predictive modeling, helping hospitals allocate resources wisely.
- Social Media Analytics: Understanding audience engagement patterns improves marketing strategies.
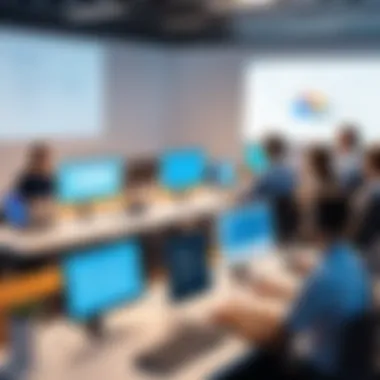
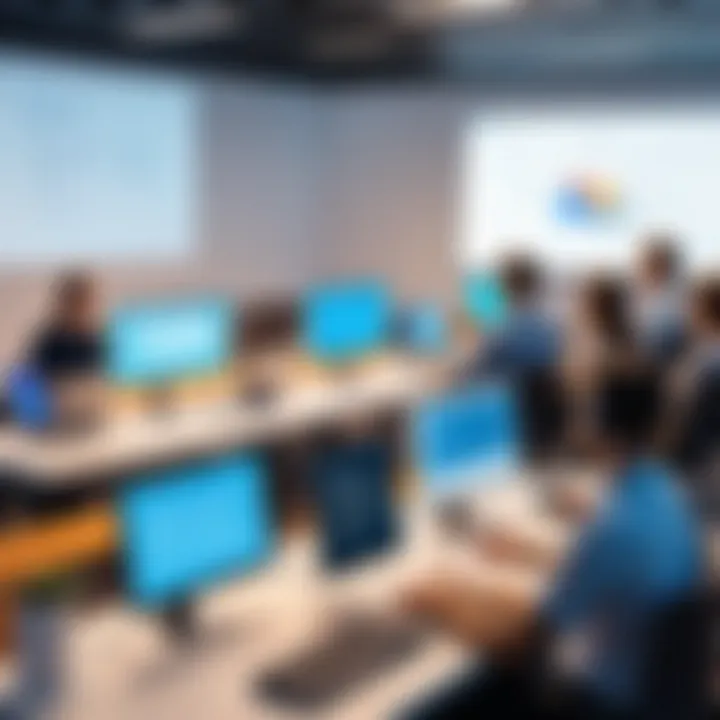
Engaging with these courses provides an opportunity to work on projects that mimic real-world problems. This hands-on experience solidifies the learning and prepares students for practical application in their careers.
"The only way to do great work is to love what you do." – Steve Jobs
Harnessing the outcomes of free data science courses on Coursera can lead to significant career advantages. As learners gain skills, they can apply those skills in various contexts, enhancing their employability and practical understanding of data in their field.
Challenges of Online Learning
Navigating the world of online education, particularly in a field as dynamic as data science, presents its own unique set of challenges. While the allure of free courses on platforms like Coursera is hard to resist, learners must acknowledge potential difficulties that can arise in this virtual learning environment. Recognizing these challenges is essential, as understanding them can lead to more effective learning habits and ultimately, greater success.
Self-Motivation and Discipline
In the digital classroom, self-motivation is the engine that keeps the wheels turning. Without a structured timetable like a traditional classroom, it can be all too easy to get sidetracked. Imagine this: You’ve got a course lined up on data visualizations, but then Netflix drops a new series, and before you know it, the course material collects digital dust.
To combat this, setting personal milestones can be helpful. Begin by breaking the course into manageable chunks and assigning deadlines. Making a study schedule and sticking to it can instill a sense of discipline. Use tools like Google Calendar to set reminders about upcoming lessons or project due dates. It’s not just about completing the course; it’s about building habits that will serve you well beyond the classroom.
"Discipline is the bridge between goals and accomplishment." - Jim Rohn
In the end, being proactive goes a long way. Self-disciplined learners are likely to enjoy a more fruitful educational journey.
Navigating Course Resources
Once you're in the thick of things, figuring out how to maneuver through course resources can be daunting. Each course on Coursera has its own layout and flow, which can feel a bit like dashing through a maze with no clear direction. It’s crucial to familiarize yourself with the platform's features early on.
Most courses offer video lectures, quizzes, and project work, but the arrangement may vary. Make it a point to explore all available resources at the start. Check forums for discussions—a hidden treasure trove for insights and tips from peers who have completed the course.
Consider these tips:
- Look at the syllabus thoroughly: This will help you understand what to expect and when to tackle each subject.
- Take advantage of supplementary materials: Many instructors include additional readings or videos that can enhance comprehension.
- Engage with peer reviews: Reading others’ perspectives on assignments can provide valuable learnings and may spark ideas for your own work.
Understanding how to effectively navigate and utilize course resources not only enhances learning quality but can also foster connections with fellow learners, enriching the experience considerably.
Comparative Analysis
In an era where online learning has gained immense traction, analyzing the distinction between free and paid courses becomes crucial for learners seeking to maximize their educational investments. This section explores how we can discern the value of free data science courses against their paid counterparts. Understanding these differences not only informs choices but enlightens learners on what to expect and how to leverage the resources available effectively.
A comparative analysis can reveal several factors that merit consideration:
- Content Quality: Are the materials comprehensive? Do they cover the latest trends and tools in data science? Paid courses often provide richer content curated by experienced professionals. In contrast, free courses might stick to fundamentals but can still be beneficial for beginners.
- Instructor Experience: The backgrounds of instructors can greatly impact the learning experience. Look into who is teaching. Paid courses generally come with qualified instructors who bring wealth of knowledge. Free course instructors might be less established, but many still share valuable insights.
- Course Duration and Depth: Paid courses might offer extended content, deeper dives into specialized topics, and additional modules, while free courses often have shorter formats, focusing on key areas.
- Networking Opportunities: Some paid courses provide networking functions, allowing students to interact with professionals and peers. By contrast, free courses sometimes lag in community aspects, limiting learner interaction.
- Certificates: A key difference comes down to certifications. Paid courses often award recognized credentials that can enhance your resume, while many free options do not offer such certificates.
"Education is the passport to the future, for tomorrow belongs to those who prepare for it today."
As much as paid courses can provide more structured, in-depth, and interactive learning, the key assertion here is that free courses should not be dismissed. They can serve as an introduction, a supplement, or even a standalone choice for those pursuing self-education in data science.
Free vs. Paid Courses
When weighing options between free and paid data science courses, it’s important to note that each has its strengths and weaknesses. Free courses offer accessibility and ease of entry without a financial commitment, making them ideal for those who are unsure about whether to pursue data science seriously. They usually cover basic concepts, making them perfect for new learners to gauge their interest in the field.
On the other hand, paid courses often come packed with features like personalized feedback, more detailed resources, and better networking possibilities. Here's a further breakdown:
- Commitment Level: Free courses can sometimes feel like an afterthought, with less commitment to student success. Paid courses may enforce stricter deadlines and more robust assessment methods.
- Resource Accessibility: In a free setting, access can be sporadic, and the support might be limited. Paid courses generally include dedicated support channels.
- Learning Environment: The atmosphere may greatly differ; a structured paid course can foster better concentration compared to the self-directed nature of free courses.
Course Reputation and Reviews
Reputation plays a significant role in the effectiveness and perceived value of a course. Platforms like Coursera rank highly among learners, but not all courses receive equal praise.
When considering a course, it's beneficial to look at:
- User Reviews: Feedback from past or current learners can provide insights into the course content, pacing, and overall effectiveness. This can help gauge if the course meets your needs.
- Accreditation and Collaborations: Courses backed by well-known universities or companies, such as Stanford or Google, tend to hold more weight. Recognizing endorsements can lend credibility and often correlates with higher quality.
- Community Discussions: Engaging with other learners can offer additional perspectives. Forums on platforms like Reddit or specialized education groups on Facebook often highlight these discussions.
Integrating Data Science Skills into Career Paths
In the modern job landscape, integrating data science skills into career paths can be a game changer. With data becoming a cornerstone of decision-making across sectors, having a solid grasp of data science not only enhances a professional's toolkit but also opens numerous doors for career advancement.
Data science isn't limited to specific roles; instead, it transcends various industries and needs. Whether it's finance, healthcare, retail, or technology, every field can benefit sharply from professionals adept in data analysis and interpretation. As the demand for data-driven insights accelerates, understanding the nuances of data science allows individuals to stay ahead in their careers.
Data Science in Various Industries
Data science finds its place in a myriad of industries, making it a versatile and valuable skill set. Here are a few sectors where data science is making waves:
- Healthcare: In this field, data science plays a critical role in improving patient outcomes. Hospitals use it for predictive analytics to foresee trends in patient readmission. Furthermore, it aids in tailoring personalized treatment plans based on data gathered from previous treatments.
- Finance: The finance world thrives on data. Banks and financial institutions use data science for risk assessment, fraud detection, and market trend analysis. Those skilled in data science can help in creating algorithms that predict stock behavior or consumer spending patterns.
- Retail: Retailers are heavily investing in data science to enhance customer experiences. From inventory management to targeted marketing campaigns, data insights help in crafting strategies that resonate with consumer behavior patterns.
- Technology: Tech companies rely on data for product development and customer service optimization. Data visionaries here might create algorithms to improve machine learning applications or strengthen cybersecurity defenses.
The takeaway? No matter the industry, having data science skills puts you in a better position to leverage data for strategic advantage.
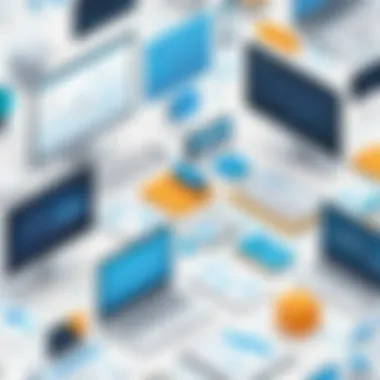
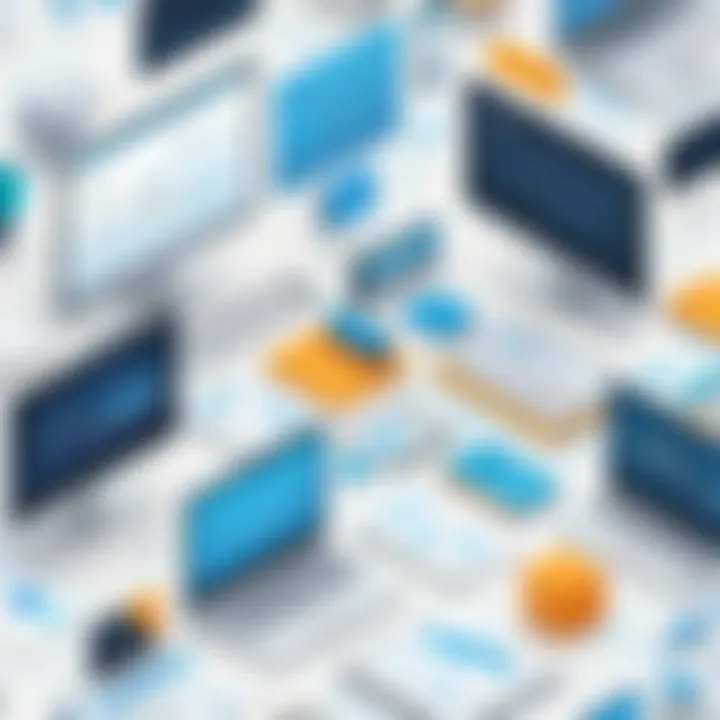
Employment Opportunities for Learners
As organizations continuously seek skilled data practitioners, the job market brims with opportunities for those who have invested time in learning data science. Here are some roles that actively demand data science skills:
- Data Analyst: This entry-level role focuses on interpreting and analyzing data to inform business decisions. Many firms are seeking candidates with analysis experience to report trends and patterns.
- Data Scientist: A slightly more advanced position, data scientists create predictive models and recommend actionable insights based on complex analyses. This role is crucial in almost all sectors.
- Machine Learning Engineer: With a focus on creating algorithms that make automated decisions, this role is ideal for those with programming skills alongside data science expertise.
- Business Intelligence Analyst: This role revolves around analyzing data to help organizations make informed decisions and strategies. A good fit for someone who is detail-oriented and has strong analytical abilities.
Here’s a key point to remember:
Investing in free data science courses can furnish you with the necessary skills, paving the path toward these lucrative career opportunities.
The timeline for entering one of these roles is often shorter than anticipated, particularly when guided by comprehensive, well-structured courses found on platforms like Coursera. As a last note, the demand for data skills is not expected to plateau soon; instead, it is anticipated to grow, promising a bright horizon for learners willing to adapt and evolve.
Feedback and Community Support
The realm of data science education is not just about consuming content; it's equally about interaction and feedback. Engaging with communities and receiving constructive critiques can substantially enhance the learning experience. Feedback and community support serve as vital components in mastering the nuances of data science, especially in an online environment where self-paced learning can sometimes feel isolating.
Engaging with Course Communities
When you enroll in a free data science course on Coursera, you step into a vibrant ecosystem of learners and instructors. These online forums, discussion boards, and social media groups can be treasure troves of insights. Connecting with course communities allows you to share experiences, ask questions, and even collaborate on projects.
- New Perspectives: Engaging in discussions often brings to light different viewpoints and approaches to data problems that you might not have considered on your own.
- Networking Opportunities: This engagement can lead to valuable connections—meeting peers or industry professionals could open doors to job opportunities or collaborative projects.
- Real-Time Support: Whether you're stuck on a coding problem or need guidance on applying a concept, community members often offer quick responses, facilitating a smoother learning journey.
Taking an active role in these communities not only enriches your learning but also fosters a sense of belonging. You realize you are not alone in this journey; others are facing similar challenges.
Importance of Peer Feedback
Peer feedback in an educational context is like having a fresh set of eyes on your work. It offers an essential layer of validation and critique that can be incredibly useful in grasping complex concepts.
- Constructive Critiques: Receiving feedback from fellow learners can highlight areas for improvement. Unlike assessments from lecturers that may be scarce, peer evaluations are more frequent and can be tailored to your current level of understanding.
- Enhancing Skills: This process of giving and receiving feedback cultivates critical thinking and improves your ability to articulate your thoughts. You learn not just to receive, but to provide guidance as well.
- Motivation Boost: Positive feedback from peers can serve as a powerful motivator. Knowing that others recognize your effort builds confidence and encourages further engagement with the material.
In short, embracing feedback and community interactions can elevate your learning experience. They bridge knowledge gaps that may exist in self-study while enriching your skill set for the real world.
"Feedback is the breakfast of champions."
This quote encapsulates the essence of why engaging with community support is indispensable. The willingness to seek out and apply feedback can transform a rudimentary understanding of data science into a robust, practical skill set.
Future of Data Science Education
The landscape of data science education is shifting rapidly, influenced by technological advancements and the ever-changing job market. This section will delve into the significance of understanding the future of data science education, especially in the context of Coursera’s free courses that cater to a broad audience. By grasping these key elements, individuals aspiring to enhance their careers can better position themselves in a competitive field.
Evolving Trends in Data Science Learning
In recent years, the terrain of data science learning has evolved remarkably. Several trends are emerging that warrant attention:
- Blended Learning Approaches: Many educational platforms are integrating both online and offline learning resources. This hybrid approach caters to different learning styles and enhances skill acquisition.
- Microlearning: Bite-sized learning modules have gained traction. These focused lessons allow learners to grasp complex concepts in a manageable time frame, making the learning process less daunting.
- Real-World Applications: Courses are increasingly designed with practical application in mind. Enrollers can expect hands-on projects that mirror real-world challenges, fostering deeper understanding and retention of material.
One major shift is the focus on collaborative learning environments. In the digital age, community interaction plays a crucial role. Learners now can engage with peers from around the globe, share insights, and collaborate on projects, creating a richer educational experience.
"Learning is a treasure that will follow its owner everywhere." – Chinese Proverb
This adage rings especially true in data science today, as collaboration fosters innovation and skill development, crucial for navigating the complexity of practical data applications.
Potential for New Course Offerings
As data science continues its march into new industries, opportunities for new course offerings become aplenty. Here are vital points to consider:
- Market Demand: Industries are increasingly needing specialized skills like machine learning and artificial intelligence. Courses focusing on these emerging fields will become staples in data science education.
- Interdisciplinary Approaches: Knowledge in data science can be highly beneficial across different sectors—healthcare, finance, and marketing. Future courses are likely to integrate data science with these fields, offering tailored education for specific professions.
- Customization and Personalization: Adaptive learning technologies that assess a learner's existing skills to curate a personalized curriculum will shape future offerings. This way, learners receive content that aligns with their career aspirations and current expertise, steering away from a one-size-fits-all approach.
An increase in partnerships between educational institutions and tech companies may also pave the way for cutting-edge content. As these alliances often result in courses developed directly from industry needs, they ensure that learners are equipped with relevant and timely skills.
In summary, the future of data science education looks promising, especially with platforms like Coursera offering flexible, accessible, and continually updated resources. By staying informed of evolving trends and exploring the wealth of new course offerings, learners can decisively enhance their skill set and career potential in this dynamic field.
Finale
In summing up the exploration of free data science courses available on Coursera, it’s clear that these offerings present a golden opportunity for anyone looking to dive into the field of data science without burning a hole in their pocket. Here are a few essential elements to consider:
- Variety of Courses: Coursera features a diverse array of courses focusing on various aspects of data science, from machine learning to statistical analysis. This variety allows learners to tailor their education based on specific interests or career goals.
- Quality Education: Despite being free, many of these courses are created by reputable institutions or instructors with significant experience in the field. This means learners are not sacrificing quality for affordability.
- Skill Development: The courses are designed not just to impart theoretical knowledge, but also to equip learners with practical skills applicable in real-world scenarios. This blend of skill and theory is vital for those aiming to make a mark in the data science space.
As you reflect on these points, it's worth noting that the journey in data science is ongoing. There’s always something new to learn; whether it’s a new tool, a technique, or an updated trend in the industry.
Summarizing Key Points
To recap, here are the key highlights of this article:
- Accessibility: Free courses on Coursera break down barriers to education.
- Course Diversity: A range of topics and structures to fit different learning styles.
- Skill Acquisition: Courses' focus on practical applications empowers learners.
- Institutional Credibility: Many courses are offered by trusted educational entities.
- Adaptability: Learners can tailor their paths based on personal interests and career aspirations.
Encouragement to Explore Free Resources
"Education is the most powerful weapon which you can use to change the world."
- Nelson Mandela
With the redefining nature of data in today’s world, leveraging such free resources could set you apart in a crowded job market. So why wait? Go ahead, explore those free courses on Coursera, and unlock the potential that lies within you.