Exploring MDM Master: A Comprehensive Guide
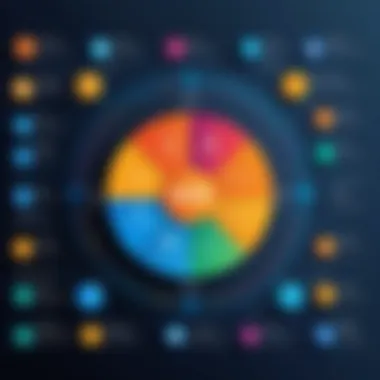
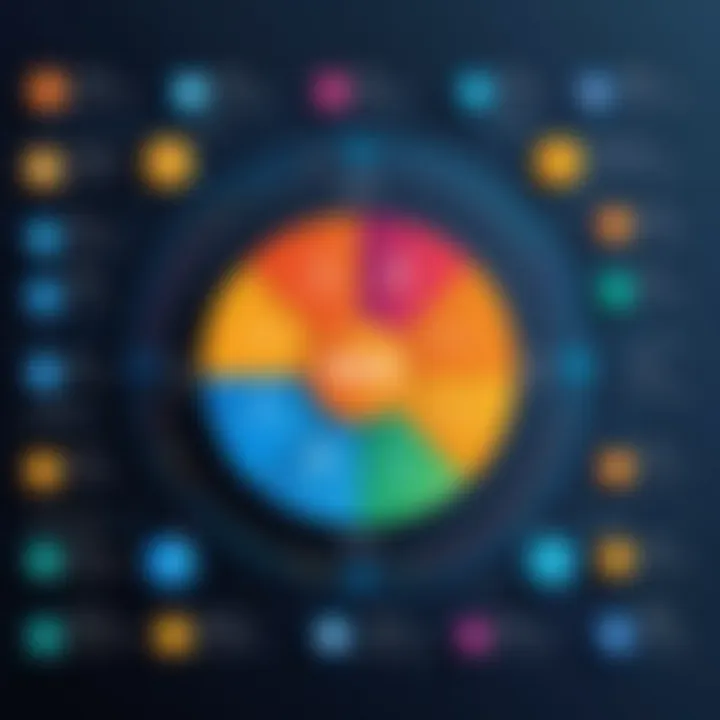
Intro
Master Data Management (MDM) has become a pivotal factor in the success of data governance strategies across various industries. The understanding and management of master data are essential to enable organizations to operate efficiently, make informed decisions, and optimize customer experiences. Central to this is the concept of MDM Master, which plays a significant role in ensuring the consistency, accuracy, and accountability of vital data entities.
In an era where data is generated and consumed at an unprecedented rate, the challenge has risen to not only capture this data but also organize and utilize it effectively. The need for clarity and precision in data management is more evident than ever. This guide will provide insights into MDM and its core component, MDM Master. It will highlight key features, performance evaluations, best practices, and common challenges faced during implementation.
Understanding MDM and MDM Master is critical for professionals across IT and data-driven sectors, as it informs the choices made around software solutions and data strategies. Therefore, diving into this topic paves the way for organizational success and innovation.
Key Features
Overview of Features
MDM Master technology is designed to provide a framework for managing data entities consistently and accurately across various systems and applications. The core features often include:
- Data Integration: MDM Master consolidates data from multiple sources, creating a single source of truth.
- Data Quality Management: This feature ensures the accuracy, validity, and consistency of master data.
- Hierarchy Management: Managing complex relationships between data entities is crucial for effective data governance.
- Workflow Automation: Streamlining data management processes enhances efficiency and reduces errors.
- Compliance Management: Helps organizations maintain regulatory compliance through accurate data practices.
The combination of these features equips organizations to tackle data silos and enhances the overall quality of decision-making.
Unique Selling Points
The unique selling points of MDM Master solutions differentiate them from other data management systems:
- Scalability: MDM Master solutions can grow with an organization, accommodating increasing data volumes.
- Customization: Tailoring the MDM Master solutions to fit specific business needs is often an integral aspect.
- Real-Time Access: Users benefit from instant access to accurate master data which is crucial in today’s fast-paced environment.
- Robust Reporting Capabilities: Advanced analytics and reporting ensure stakeholders are well-informed for strategic planning.
These properties make MDM Master an invaluable asset for organizations that prioritize data integrity and effective management.
Performance Evaluation
Speed and Responsiveness
One vital aspect to consider is the speed and responsiveness of MDM Master systems. Timeliness of data is critical for decision-making. Systems need to not only ensure quick retrieval of data but also maintain responsiveness while handling large volumes of transactions. Performance metrics can be regularly monitored to ensure that the system meets organizational demands.
Resource Usage
Lastly, examining resource usage is essential. Resource optimization contributes to cost-efficiency. An effective MDM Master solution should make use of available resources without overburdening IT systems. This is crucial for maintaining operational agility and a scalable data infrastructure.
Effective data management is not just a requirement but a competitive advantage in today's marketplace.
The insights gained from performance evaluation guide organizations in fine-tuning their MDM implementations for maximum impact. Through understanding the features and evaluating performance, organizations are better positioned to enhance their data strategies, streamline operations, and meet stakeholder needs.
Prolusion to Master Data Management
In the ever-evolving landscape of data management, the concept of Master Data Management (MDM) has emerged as a critical practice for organizations seeking to streamline their data handling processes. This section introduces the fundamentals of MDM, setting the stage for understanding its intricate components and their significance in managing data effectively. Organizations today are inundated with vast amounts of data. Without a robust framework to manage this data, including ensuring its accuracy and consistency, organizations may struggle to leverage it effectively in their decision-making processes.
Definition and Overview of
Master Data Management can be defined as a set of processes, policies, and tools used to ensure the uniformity, accuracy, and accountability of the organization's master data assets. Master data typically includes essential business entities such as customers, suppliers, products, and locations. The overarching goal of MDM is to create a single, trusted view of such data across the organization.
By establishing a clear definition of what constitutes master data, organizations can avoid ambiguity and ensure all stakeholders have a mutual understanding. MDM encompasses various activities, including data governance, data integration, and data quality management. It acts as a unifying framework, allowing disparate data sources to be harmonized and providing a reliable foundation for other data management initiatives.
Importance of Master Data in Organizations
Organizations increasingly recognize the vital role that master data plays in their operations. Having accurate and consistent master data is critical for several reasons:
- Improved Decision Making: Reliable master data enables better analytics and reporting, leading to more informed strategic choices.
- Operational Efficiency: Streamlined processes can be achieved when there is a single source of truth, reducing redundancies and errors in data handling.
- Regulatory Compliance: Maintains data integrity, which is crucial for adhering to various compliance frameworks and minimizing risk.
- Enhanced Customer Experience: Accurate customer data leads to personalized experiences, boosting customer satisfaction and loyalty.
"The strength of an organization's decisions is directly proportional to the quality of its data."
In essence, the importance of Master Data Management cannot be overstated. It serves as the backbone of an organization's data strategy, ensuring that all levels of the organization are well-informed and equipped to operate efficiently and effectively. As we delve deeper into the subsequent sections of this article, we will explore how MDM operates, the challenges organizations face, and best practices for implementation.
Master Explained
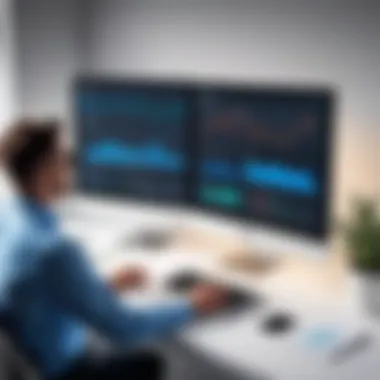
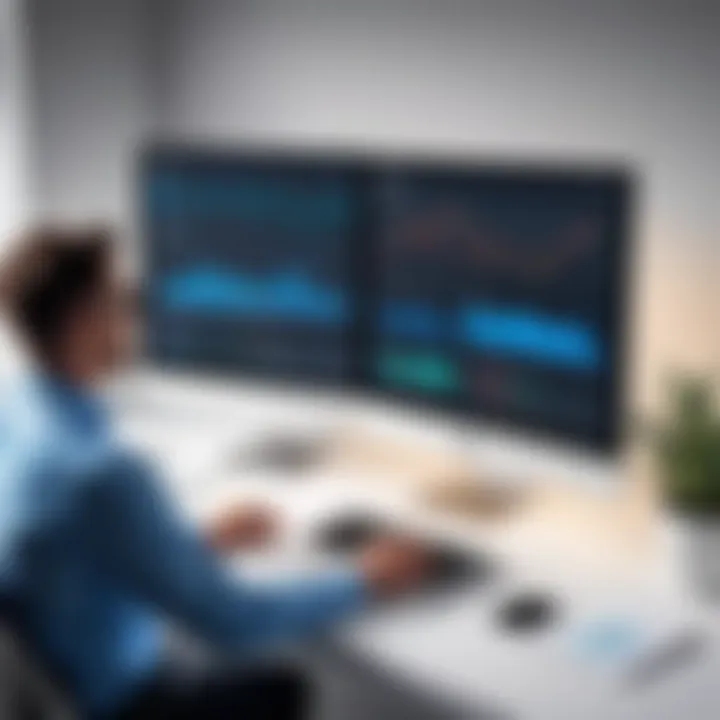
Master Data Management (MDM) is an essential framework that organizations utilize to ensure the consistency and accuracy of their master data. Understanding MDM Master is paramount as it directly impacts how companies handle their critical data assets. The necessity for robust MDM systems has escalated due to complex business environments and increasing regulations. When properly implemented, MDM Master creates a single, authoritative source of truth for all vital data. This section breaks down the fundamentals of MDM Master, focusing on its definition and key components.
Understanding Master
MDM Master refers to the specific strategies and systems designed to manage an organization’s master data. Master data includes key variables, such as customer information and product details, that are essential for daily operations. Without effective MDM, businesses run the risk of data silos where information is duplicated or inconsistent across different departments. This inconsistency can severely hinder decision-making processes and operational efficiency.
The role of MDM Master extends beyond mere data management. It fosters a culture of data stewardship and accountability, promoting better communication across departments. As industries evolve, understanding MDM Master becomes critical for staying ahead in a data-driven environment.
Key Components of Master
The framework of MDM Master consists of several key components that organizations must consider to effectively harness master data. These include:
- Data Integration: MDM Master integrates data from various sources, ensuring that all information is consolidated into a singular source. Integration reduces the chances of discrepancies and redundancy.
- Data Quality Management: Maintaining the quality of master data is vital. This involves continuous monitoring and cleansing processes to remove inaccuracies and incomplete records.
- Data Governance: Establishing clear data governance practices involves defining roles and responsibilities regarding data usage and management. This practice protects sensitive information and ensures compliance with relevant regulations.
- Data Lifecycle Management: This component oversees the lifecycle of data from creation to retirement. It ensures that data remains relevant and useful throughout its existence.
In summary, MDM Master is not just about storage; it's about strategic management of an organization's most valuable data. The benefits of understanding and implementing MDM Master lead to more informed decision-making, improved business processes, and enhanced overall performance.
"Effective Master Data Management is crucial for any organization looking to thrive in today's data-rich landscape."
By integrating these components into their MDM strategies, organizations can forge a robust framework that not only addresses current data challenges but also prepares them for future demands.
The Role of in Data Governance
Master Data Management (MDM) serves a pivotal role in data governance by establishing a disciplined approach to managing critical data assets. It ensures that there is a single, accurate version of information across the organization. Data governance encompasses policies, procedures, and standards that manage the flow and integrity of data, making MDM an essential component. This section explores how MDM enhances data quality, consistency, and regulatory compliance.
Data Quality and Consistency
Maintaining high data quality is fundamental for organizations seeking to leverage data as a strategic asset. MDM directly contributes to improved data quality by providing a structured framework for data management. Through processes like data cleansing, integration, and synchronization, organizations can eliminate redundancies and inaccuracies in their data.
When data inconsistencies arise, they can lead to confusion and misinformed decisions. MDM solves this by enabling master records that serve as the authoritative source for business data. Each record is maintained through a systematic process, verifying its accuracy against predefined criteria.
Organizations benefit when data is consistent. For example:
- Improved Decision-Making: Consistent data allows for reliable insights, leading to better strategic choices.
- Enhanced Customer Experience: Accurate customer data helps tailor marketing and support efforts.
- Reduced Operational Risks: Less inconsistency means fewer errors in transactions and operations.
Compliance and Regulatory Aspects
Navigating compliance and regulatory requirements can be intricate for businesses, especially in industries such as finance, healthcare, and retail. MDM supports compliance efforts by providing a robust framework for managing sensitive data. It helps maintain a clear audit trail and report on data lineage, which is crucial for meeting legal standards.
With MDM, organizations can:
- Ensure Data Accuracy: Accurate master data protects against regulatory penalties that can arise from incorrect reporting or disclosures.
- Facilitate Audits: An organized data governance model means that compliance audits can be conducted more efficiently and effectively.
- Protect Customer Information: MDM helps in safeguarding sensitive data, thus adhering to privacy laws such as GDPR and HIPAA.
By aligning MDM with governance strategies, businesses create a clear pathway to meeting compliance requirements.
"MDM is not just about managing data; it is about managing risks and ensuring that regulatory obligations are met seamlessly."
Ultimately, the integration of MDM into data governance schemes not only defends against potential compliance issues but also builds a sustainable data management culture.
Implementation Strategies
Master Data Management (MDM) implementation strategies are crucial in ensuring that organizations can effectively manage their data assets. Poorly executed MDM can lead to data silos, inconsistencies, and hindered business operations. Hence, a well-defined strategy streamlines processes, enhances data quality, and fosters data stewardship within organizations.
When discussing MDM implementation, it is important to consider the following elements:
- Alignment with Business Goals: Any MDM strategy should correlate closely with the broader objectives of the organization. Understanding how MDM impacts customer experience, operational efficiency, and regulatory compliance aids in prioritizing tasks.
- Stakeholder Involvement: Engaging stakeholders from various departments can facilitate smoother adoption. Their input can help tailor the MDM strategy to meet different data needs across the organization.
- Change Management: Implementing MDM often requires changes in processes and culture. Proper change management strategies that include training programs and ongoing support help mitigate resistance.
The benefits of having a structured MDM implementation strategy include:
- Improved Data Quality: A clear approach to data governance results in more accurate and consistent data.
- Enhanced Decision Making: Access to reliable master data allows for better insights and informed decision-making.
- Regulatory Compliance: A stable and transparent MDM setup aids in meeting compliance requirements effectively.
Step-by-Step Approach to Implementation
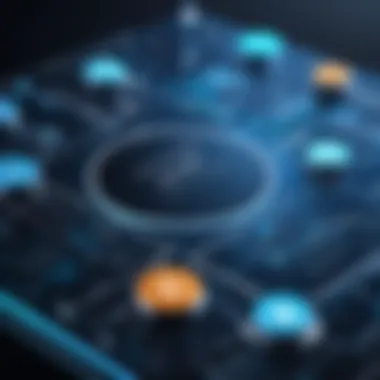
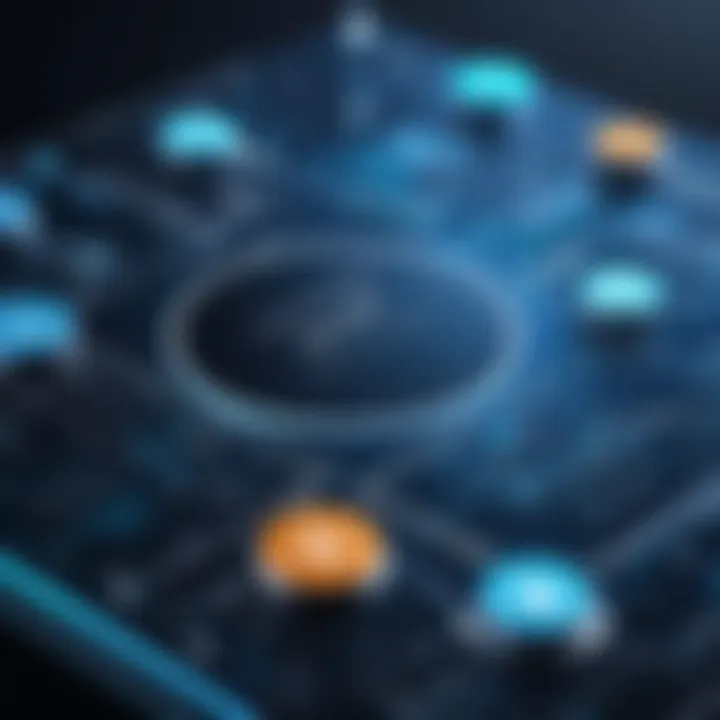
A step-by-step approach to MDM implementation provides a roadmap for organizations. This method reduces errors and conflicts during the deployment phase, making the entire process more manageable. Below are essential steps:
- Assess Current State: Evaluate existing data systems, identify gaps and establish a baseline for where you currently stand. A comprehensive audit helps offer insights into the challenges that need addressing.
- Define Objectives: Clearly articulate what you want to achieve with MDM. Are you looking to improve data quality, unify information, or enable analytics? Goals should be SMART—specific, measurable, achievable, relevant, and time-bound.
- Select the Right Team: Form a project team with members from IT, business units and data governance. A diverse group can ensure all perspectives are taken into account.
- Choose MDM Tool/Technology: Select appropriate technology that fits your organizational size, budget, and specific need. The right resources can help facilitate seamless integration and foster user adoption.
- Data Modeling: Create a model that defines how data will look in the new setup. This includes data templates, hierarchies, and defining key attributes.
- Develop a Governance Framework: Establish guidelines for managing data quality, data stewardship, and data ownership. Ensuring long-term data integrity requires a robust governance model.
- Implement and Iterate: Roll out the MDM system, then continuously monitor effectiveness and gather feedback. Iteration based on lessons learned fosters improvements.
Choosing the Right Tool
Choosing the right MDM tool requires careful consideration and alignment with organizational needs. Key factors to evaluate include:
- Scalability and Flexibility: The tool should be capable of adapting to your organization's changing data requirements.
- Integration Capabilities: Look for interoperability with existing systems. A tool that easily connects with current technology will reduce implementation time and costs.
- User-Friendly Interface: A tool with an intuitive interface simplifies onboarding. Employees are more likely to embrace a system they find easy to use.
- Cost: Evaluate the total cost of ownership, not just the upfront expense. Consider factors like licensing, maintenance, and potential hidden costs.
- Vendor Support: Assess the support provided by the vendor. Reliable support can be critical for troubleshooting and ensuring the smooth operation of the MDM tool.
MDM tool selection is a pivotal decision that can influence the entire MDM initiative. Allocating sufficient time and resources to choose wisely can pay off in terms of enhanced data management and governance.
Common Challenges in
Master Data Management (MDM) is essential for organizations aiming for data accuracy and consistency. However, the road to effective MDM is fraught with challenges. Understanding these challenges provides insight into why MDM is crucial in today’s data-driven environment. Each challenge impacts the overall quality of the master data and, subsequently, the decisions made by stakeholders. Addressing these challenges can lead to improved efficiency and reliability in data management processes.
Data Silos and Inconsistencies
Data silos refer to isolated pockets of data which often reside in different departments or systems within an organization. These silos can give rise to significant inconsistencies in data quality. When particular departments configure their data systems independently, it results in varied data formats, definitions, and standards. This situation can hinder unified decision-making across the organization.
The importance of addressing data silos cannot be overstated. The lack of cohesion leads to duplicated efforts, where teams may unknowingly be working with outdated or incorrect data. By fostering a holistic approach to data integration, organizations can reduce these silos. Consider implementing centralized data repositories or data lakes that consolidate information from various sources, thus mitigating inconsistencies.
Scalability Issues
As organizations grow, their data management needs evolve. Scalability becomes critical in ensuring that MDM systems can adapt accordingly. Many traditional MDM solutions are built on legacy systems that may not support the required volume or velocity of data in a scaling environment. This can lead to performance issues, causing delays and impacting the overall effectiveness of data management.
To address scalability issues, organizations must choose MDM solutions that accommodate growth. Cloud-based MDM services often provide better scalability by allowing organizations to adjust resources as needed. Furthermore, adopting a modular architecture can facilitate easier updates and expansions, allowing systems to integrate new data sources seamlessly.
Ensuring that your MDM strategy is scalable is as vital as the initial implementation itself. The wrong choice can stifle growth and hinder data utility.
In summary, recognizing the common challenges in MDM—data silos and scalability issues—provides a pathway towards more effective data governance. By implementing strategies to overcome these challenges, organizations can realize the full potential of their master data initiatives.
Best Practices for Effective
Implementing best practices in Master Data Management (MDM) is crucial for organizations looking to optimize their data strategies. Effective MDM can significantly enhance data quality, streamline operations, and improve overall decision-making processes. By adhering to proven strategies, companies can mitigate risks associated with data management and ensure that their master data remains accurate and accessible.
Establishing Clear Data Governance Policies
Data governance policies serve as the backbone of effective MDM. These policies define the rules and procedures for how data is managed and utilized across the organization. Establishing clear policies helps to regulate data usage, ensuring that all stakeholders are on the same page when it comes to data responsibility.
- Assign Roles and Responsibilities: Clearly defined roles ensure accountability. Assign data stewards and decision-makers within the team, who understand the importance of maintaining data integrity.
- Develop Standards: Implement naming conventions, data formats, and quality metrics. This helps in standardizing data across departments and systems, leading to better data consistency.
- Establish a Data Lifecycle Management: A robust process for data creation, maintenance, and deletion reduces data clutter and optimizes data usage.
These components not only facilitate compliance with regulations but also promote a culture of data stewardship within the organization. By fostering accountability, organizations can achieve a higher level of data quality, resulting in more informed decision-making.
Regular Data Audits and Cleansing
Routine data audits and cleansing are essential for maintaining the health of master data. Regular checks help organizations identify discrepancies and data quality issues early on. Ignoring these practices can lead to persistent problems that escalate and complicate data management over time.
- Schedule Routine Audits: Conducting regular audits of data sets helps in identifying duplicates, inaccuracies, and outdated information. This is essential to keep the data relevant and trustworthy.
- Implement Data Cleansing Tools: Utilize software tools that automate the cleansing process. These tools can help in regular maintenance and ensure that the data stays accurate over time.
- Engage in Continuous Improvement: Establish a feedback mechanism that allows users to report data issues. Continuous feedback and adjustments can dramatically improve data quality and usability.
"Regular data audits not only preserve data integrity but also enhance trust among users by proving that data management is taken seriously."
Future Trends in
Understanding the future trends in Master Data Management (MDM) is crucial for organizations that want to remain competitive in the rapidly evolving data landscape. As data continues to grow exponentially, organizations face various challenges around data governance, quality, and accessibility. Emerging technologies such as artificial intelligence and cloud-based solutions are reshaping how MDM is approached, providing new opportunities and improved efficiency. This section discusses the significance of these trends, their benefits, and the considerations necessary for successful adoption.
Artificial Intelligence and
The integration of artificial intelligence (AI) into MDM systems is a significant trend. AI can enhance data processing and analysis, improving decision-making capabilities. With AI, organizations can automate processes such as data cleansing and enrichment, reducing human error and increasing accuracy. Predictive analytics powered by AI can help identify patterns in master data, allowing businesses to glean insights that may have gone unnoticed.
Some benefits of incorporating AI into MDM include:
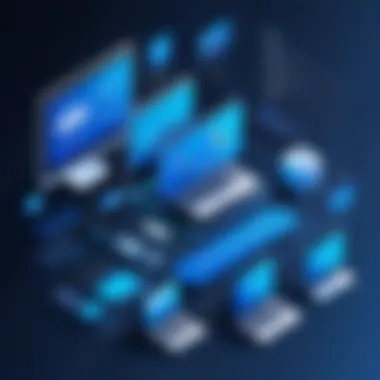
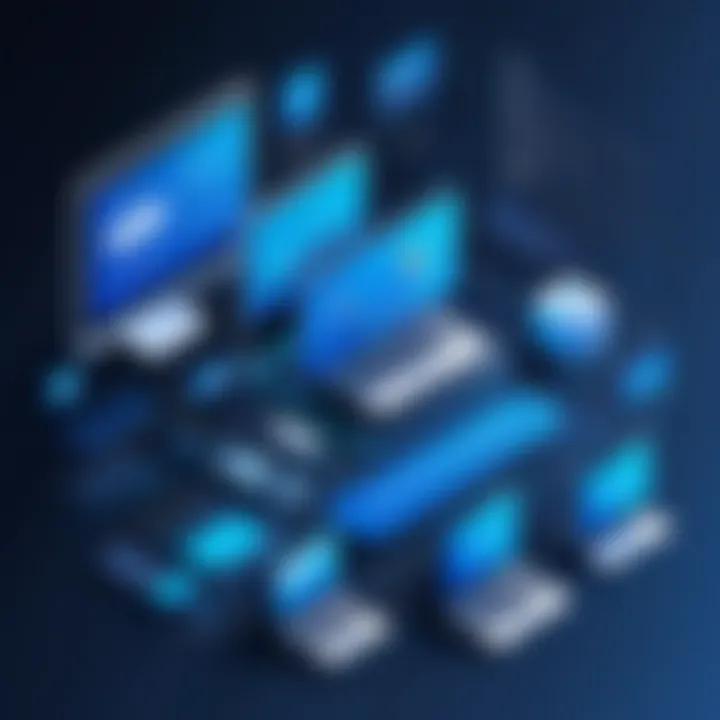
- Enhanced Data Quality: AI can continuously monitor data and flag inconsistencies automatically.
- Improved Data Governance: Organizations can enforce data policies more effectively with AI-driven tools, ensuring compliance with regulations.
- Decision-Making Support: AI algorithms can analyze large datasets much faster than humans, providing timely insights.
However, integrating AI comes with challenges. Organizations must ensure they have the right infrastructure and skillset to manage AI technologies. This requires investment and strategic planning.
Cloud-Based Solutions
The shift to cloud-based MDM solutions represents another major trend. These systems offer flexibility, scalability, and accessibility that traditional on-premises solutions may lack. Cloud-based models allow organizations to store vast amounts of data without extensive hardware investments. They can also easily adapt to changing business needs.
Key advantages of cloud-based MDM solutions include:
- Reduced Costs: By utilizing cloud services, organizations can lower their IT costs, reducing maintenance and upgrade expenses.
- Enhanced Collaboration: Cloud systems simplify access for global teams, ensuring that everyone is working with the same data.
- Rapid Deployment: Organizations can implement cloud solutions faster compared to on-premises setups, allowing quicker alignment with business goals.
Nonetheless, organizations must consider data security and compliance when transitioning to cloud-based systems. Establishing robust security protocols is vital to protecting sensitive information stored in the cloud.
In summary, emerging trends such as AI and cloud-based MDM solutions represent significant growth areas for organizations looking to optimize their master data management. Embracing these technologies can lead to improved data quality, compliance, and cost efficiency.
By staying informed about these trends, businesses can proactively enhance their MDM strategies and maintain a competitive edge in the marketplace.
Case Studies of Successful Implementation
The exploration of case studies in Master Data Management (MDM) provides tangible evidence of MDM's effectiveness, revealing how various organizations harness MDM for better data governance and performance.
Through these real-world examples, one can identify both the benefits and challenges faced by industries, which can guide efforts in implementing similar strategies.
Notably, these studies exemplify how tailored solutions address unique data management needs across various sectors, influencing decisions that lead to refined practices.
Industry-Specific Examples
Retail
In the retail sector, MDM has transformed data handling by harmonizing diverse datasets from multiple channels. Retailers face constant pressure to provide personalized experiences to consumers while maintaining operational efficiency. One significant advantage of MDM in retail is its ability to create a unified view of customer data, which, in turn, enhances customer relationship management.
Retailers like Target demonstrate effective MDM by integrating customer purchase histories with inventory data. This synchronization allows for better stock management and enhances marketing strategies. A unique feature of their approach is the use of AI-driven analytics to anticipate customer needs based on historical trends. However, the challenge lies in managing data privacy and ensuring compliance with regulations.
Healthcare
Healthcare has a distinct requirement for accurate and consistent data due to its direct impact on patient welfare. MDM plays a crucial role in consolidating patient information from various sources like hospitals, clinics, and labs. This consolidation improves the quality of care by providing healthcare professionals with a holistic view of patient data, thereby reducing errors.
An interesting characteristic of healthcare MDM is its emphasis on compliance with strict regulations, such as HIPAA in the United States. This makes the implementation of MDM not just beneficial but essential for operational viability. However, sensitive data handling presents unique security challenges that require robust protection measures to safeguard patient information.
Finance
The finance industry requires precision and accuracy in data due to regulatory scrutiny and risk management. MDM enables financial institutions to maintain a clear, accurate view of data, which is vital for compliance and decision-making. For example, banks utilize MDM to ensure customer data is consistent across various departments, thus enhancing customer service and operational efficiency.
A key characteristic of MDM in finance is its ability to support real-time data access. This feature allows for timely decision-making and risk analysis, which are crucial for the financial sector's operational health. Yet, the challenge remains to continually validate data sources to avoid risks stemming from data inaccuracies, which can have serious implications for financial reporting and compliance.
In summary, case studies in MDM not only underscore its significance across industries but also highlight the tailored strategies necessary for effective implementation.
By examining these industry-specific examples, practitioners can glean insights into MDM application, challenges to anticipate, and strategies for overcoming those obstacles.
Epilogue
Master Data Management (MDM) is crucial for organizations that seek to maintain accuracy in their data environments. As businesses generate vast amounts of data, having a clear and reliable master data strategy becomes essential. This article has presented an in-depth exploration of MDM, particularly focusing on MDM Master as a fundamental component.
To recap, the key points discussed include the definition and significance of MDM, its role in data governance, various implementation strategies, and common challenges faced by organizations. Each segment reflects the necessity of a structured approach to managing master data effectively.
Recap of Key Points
- Importance of MDM: MDM helps organizations in ensuring data consistency, which benefits decision-making processes and operational efficiency.
- Implementation Strategies: Choosing the right tools and following a systematic approach can aid in overcoming common challenges such as data silos and scalability issues.
- Best Practices: Establishing robust data governance policies and conducting regular data audits ensures that the master data remains relevant and accurate.
- Future Trends: Technologies like artificial intelligence and cloud-based solutions are transforming how MDM is approached, offering new capabilities for better data management.
By understanding these critical concepts, organizations can not only enhance their data quality but also realize the full potential of their data assets.
The Future of Master
The future of MDM Master is trending towards increased automation and intelligence. As organizations continue to pursue efficient data management practices, tools that employ artificial intelligence will be at the forefront. These tools can assist in improving data quality by identifying inconsistencies and suggesting corrections.
Cloud-based MDM solutions are also gaining popularity. They offer flexibility, scalability, and cost-effectiveness, making them attractive choices for companies of all sizes. The ability to access and manage data from various locations seamlessly will become a standard expectation in data management.
As data management continues to evolve, organizations must remain adaptable and forward-thinking to stay competitive in the digital landscape.