Data Mapping: A Critical Tool in Business Analysis
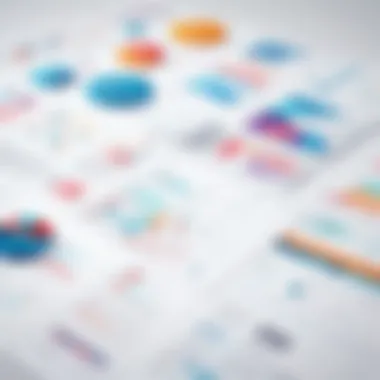
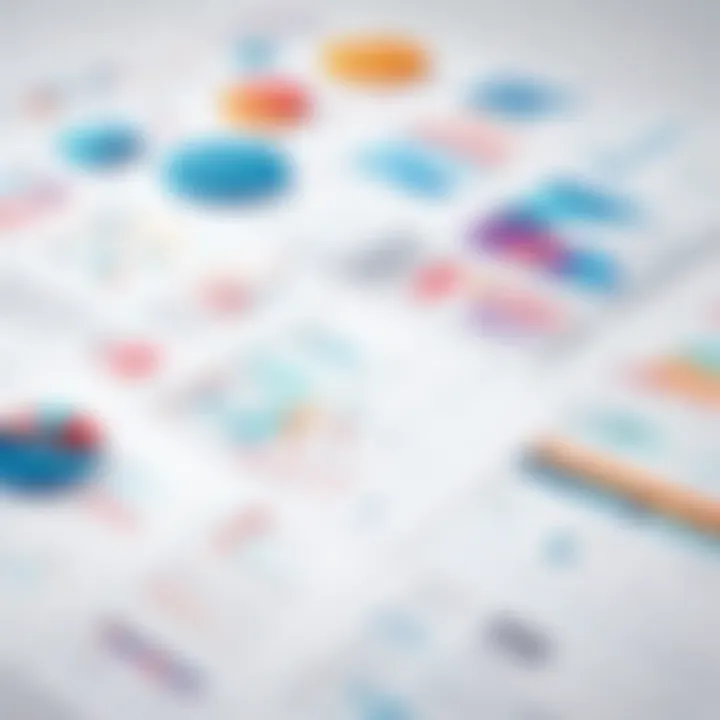
Intro
Data mapping emerges as a critical component in the domain of business analysis. It represents a systematic process utilized to connect data elements from diverse sources and systems. With organizations increasingly dependent on data-driven insights, the significance of data mapping grows substantially. This process not only aids in unraveling the complexity of data but also ensures seamless data integration and effective management strategies.
When organizations grapple with large volumes of data from various sources, the need for a robust data mapping strategy becomes apparent. Such strategies involve transforming raw data into meaningful information that can inform business decisions. Thus, understanding data mapping is not merely an academic exercise; it is a practical necessity.
Every stage of business analysis can benefit from well-executed data mapping. From identifying data sources to delivering actionable insights, data mapping plays an invaluable role. It helps in ensuring accuracy, compliance, and consistency in data, which ultimately enables organizations to perform better in todayโs competitive marketplace.
In this article, we will uncover the intricacies of data mapping, explore its methodologies, examine the benefits it brings to businesses, and discuss the skills that are necessary for successful implementation. Furthermore, we will address common challenges faced in data mapping and outline effective practices to overcome these obstacles.
Understanding Data Mapping
Data mapping plays a crucial role in business analysis, particularly in the domain of data management and integration. Understanding data mapping is essential for professionals navigating today's data-driven environment. It enables stakeholders to translate data from one format to another, ensuring a seamless transfer and alignment of information across various systems.
The advantages of data mapping extend to multiple aspects of organizational operations. By having a clear understanding of how different data elements relate to each other, businesses can achieve better data quality. The integrity of data becomes paramount as inaccuracies can propagate through systems and result in flawed analyses, ultimately hindering decision-making processes. Data mapping serves as a foundation for establishing data governance and compliance, ensuring that sensitive information adheres to regulatory frameworks while meeting organizational standards.
In addition, understanding data mapping fosters efficient data integration. As organizations often utilize heterogeneous systems, effective mapping is key to aligning diverse data sources, thereby creating a unified view of information. This is particularly valuable for businesses aiming to enhance their analytical capabilities, as accurate and synchronized data is vital for insightful decision-making.
Moreover, comprehension of data mapping concepts reduces risks associated with data migration. In instances where system upgrades or changes in infrastructure occur, a solid mapping strategy assists in mitigating potential obstacles, ensuring continuity and minimizing disruptions.
"The clarity of data relationships provided by mapping informs not only how data moves but also how it can be leveraged for strategic advantage."
Hence, traversing the landscape of data mapping involves understanding its definition and purpose. This groundwork equips business analysts with the knowledge necessary to navigate challenges while implementing best practices. As we delve deeper into the specifics, the foundation laid in this understanding will guide further exploration into effective methodologies and practical applications.
Role of a Business Analyst in Data Mapping
In the context of data mapping, the role of a business analyst is crucial. They act as the bridge between technical teams and business stakeholders, ensuring clarity and understanding in the data mapping process. A business analystโs insights into the needs and dynamics of a business enable the mapping to align more closely with business goals. Effective data mapping is not just about moving data; it involves understanding the actual requirements and ensuring that the data serves a strategic purpose.
Key Responsibilities
A business analyst undertakes several key responsibilities throughout the data mapping lifecycle:
- Requirement Gathering: The analyst must clarify what data needs to be mapped, why it's mapped, and what the end goal is. This can involve discussions with stakeholders to gather detailed requirements.
- Data Analysis: They must review existing data structures, sources, and quality. Understanding what data exists and how it relates to business objectives is fundamental.
- Creating Mapping Documentation: Analysts document the relationships between different data sources and how they should be integrated. This documentation serves as a reference for developers and data engineers.
- Collaboration with IT Teams: Business analysts work closely with IT and development teams. They must communicate clearly to ensure that technical team understands the goals of mapping.
- Testing and Validation: They play a role in testing the mapped data to ensure it meets the requirements. Any discrepancies must be addressed promptly.
These responsibilities emphasize the analystโs role in shaping the mapping outcomes to align with overall business strategies and data governance.
Skills Required
To execute their responsibilities effectively, business analysts must possess a variety of skills:
- Analytical Thinking: They need to process complex information and understand the implications of data structures.
- Communication Skills: Clear communication with both technical and non-technical stakeholders is essential to achieve mutual understanding.
- Attention to Detail: Any small errors in data mapping can have significant consequences. Analysts must ensure accuracy.
- Technical Knowledge: While not always required to code, familiarity with data architectures, databases, and mapping tools is beneficial.
- Problem Solving: The ability to identify challenges within data mapping and propose effective solutions is critical.
Data Mapping Methodologies
Data mapping methodologies are essential in structuring how organizations approach their data management tasks. These methodologies outline the frameworks within which data is identified, understood, and transformed to meet specific business needs. A clear methodology not only helps in reducing data-related errors but also significantly enhances the quality of insights derived from that data.
Top-Down Approach
The Top-Down Approach emphasizes starting with a high-level view of the data. This involves outlining the overarching goals and objectives before drilling down into the details. Organizations often prefer this method when they have a complete understanding of their long-term data strategies.
In this approach, business analysts first identify key entities and relationships within the data. For example, in a retail context, high-level categories such as customers, products, and transactions are defined. Following this, specifics are mapped to these categories, such as customer personal details and transaction history. This hierarchical structure ensures that nothing essential is overlooked during the mapping process.
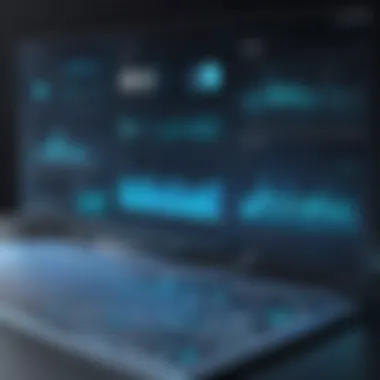
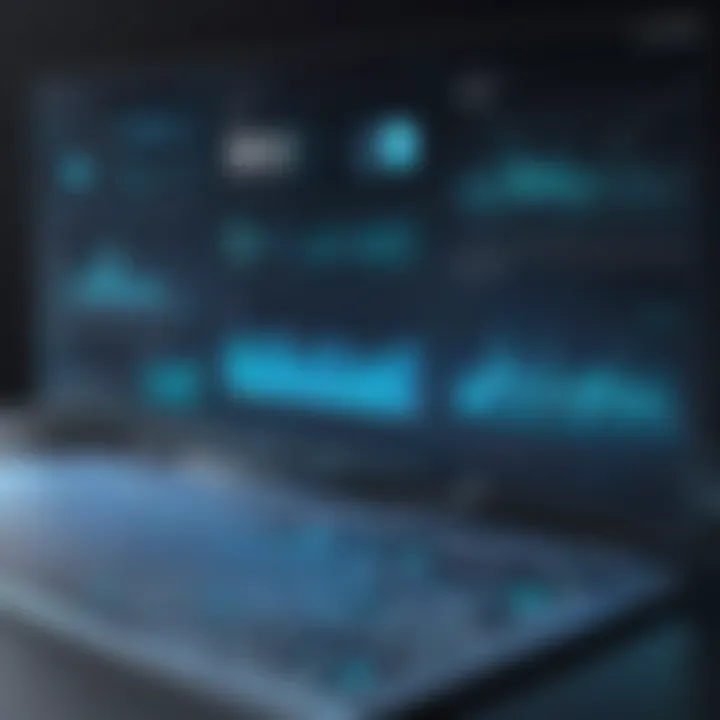
Advantages of the top-down approach include:
- Clarity in understanding overall data architecture.
- Easier identification of relationships between different data components.
- Streamlined collaboration across departments, as everyone starts from the same high-level view.
Bottom-Up Approach
The Bottom-Up Approach takes an inverse perspective by starting with individual data elements. It requires granular scrutiny of each dataset to understand its purpose and integration into larger processes. This approach works well when organizations deal with multiple, disparate data sets needing consolidation.
In practice, teams gather data from various sources, ending with a comprehensive view of how this data interacts with others. For instance, in a healthcare setting, individual patient records, treatment histories, and billing information might be gathered and then connected.
This method has its own set of benefits:
- Encourages meticulous attention to detail, often revealing hidden insights.
- Improves data accuracy by focusing on specific elements before integration.
- Aids in identifying data anomalies early in the process.
Hybrid Approach
The Hybrid Approach combines elements from both the top-down and bottom-up methods. It seeks to leverage the advantages of both to create a more robust data mapping strategy. This is particularly effective for organizations undergoing transformation or integration, where existing and new data structures need alignment.
By integrating high-level strategies with detailed data review, organizations can build a coherent data framework that is both comprehensive and precise. For instance, a company may start with overall business objectives but also continually loop in insights and adjustments from data collected at the ground level.
The Hybrid Approach offers:
- A balanced perspective, blending strategy with tactical execution.
- Greater flexibility in adapting to changing data environments.
- Enhanced capacity to spot inconsistencies during integration processes.
In summary, the choice of data mapping methodology significantly influences the effectiveness of data management initiatives in organizations. Understanding these methodologies enables business analysts to align their data strategies with overall business goals, improving decision-making and operational efficiencies.
Benefits of Data Mapping for Organizations
Data mapping plays a crucial role in enhancing organizational performance. It serves as a foundational element for effective data management across various systems and departments. By systematically organizing data, businesses can leverage their information more efficiently, which leads to significant improvements in several key areas.
Improved Data Quality
One primary advantage of data mapping lies in its ability to enhance data quality. When organizations implement proper mapping, they can identify errors or inconsistencies within their data sets. This process facilitates the detection of duplicates, misformatted entries, and missing values, all of which can skew analytical results.
Through validated data mapping processes, organizations can employ data cleansing techniques ensuring the information used is accurate and reliable. Improved data quality ultimately fosters greater trust in the insights derived from the data, allowing businesses to make informed decisions backed by solid evidence.
Enhanced Data Integration
Data mapping is essential for organizations looking to integrate disparate data sources. Often, businesses operate multiple systems, such as CRM software like Salesforce and ERP systems like SAP. Without a coherent integration strategy, data can become siloed, leading to inefficiencies. By applying data mapping, organizations can connect these systems seamlessly.
Implementing efficient data mapping allows for the consolidation of data into a unified format. This coherence greatly aids in producing comprehensive views of information across departments and systems. Consequently, the organization benefits from a more holistic outlook on its operations, enabling improved collaboration and strategy alignment.
Streamlined Decision Making
Effective data mapping further leads to streamlined decision-making processes. When data is organized and accessible, decision-makers can analyze information quickly and efficiently. This capability reduces the time spent searching for relevant data or clarifying inconsistencies, thus expediting the decision-making timeline.
Moreover, accurate and easily accessible data fosters confidence in strategic decisions. With real-time insights and a better understanding of performance metrics, businesses can react quickly to changing market conditions. Consequently, this responsiveness can provide a distinct advantage in competitive environments, allowing organizations to stay ahead.
"Data mapping is not just about data organization; it is a strategic imperative for modern businesses looking to leverage their information advantage effectively."
In summary, organizations that recognize the benefits of data mapping can greatly improve data quality, enhance data integration, and streamline decision-making processes. With these advantages, they can position themselves more effectively in the market and drive better business outcomes.
Common Challenges in Data Mapping

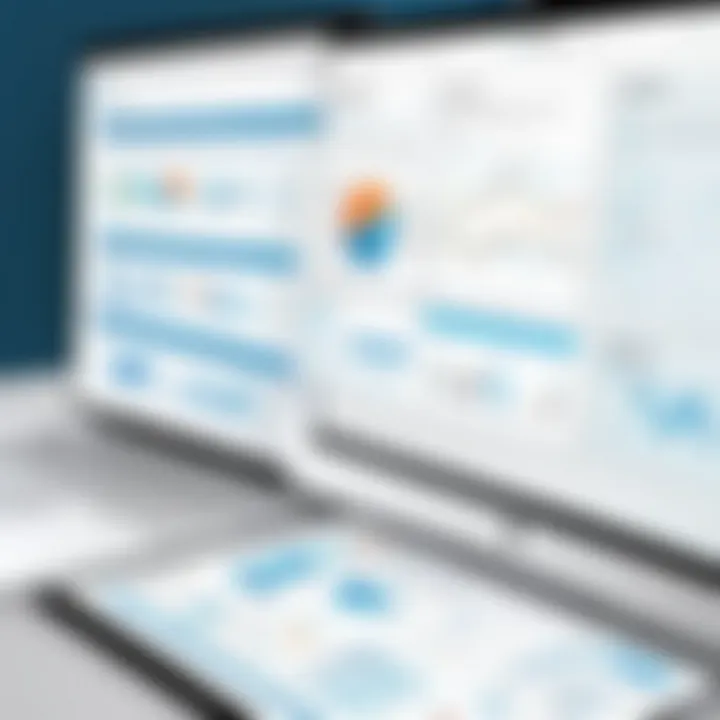
Data mapping is a complex process that, while vital for effective data management, presents several challenges. Understanding these challenges helps business analysts and IT professionals anticipate potential issues and devise strategies to mitigate them. This section outlines the key challenges faced during data mapping, focusing on data inconsistencies, complex data structures, and undefined data standards.
Data Inconsistencies
Data inconsistencies arise when data does not align across various sources. This can happen due to differences in data entry practices, outdated data sources, or a lack of alignment between organizational systems. For example, customer records may have different names, addresses, or contact details in various databases. Such discrepancies can lead to unreliable outcomes during data analysis, affecting decision-making processes.
To tackle data inconsistencies, it is essential to have clear governance on data quality. Organizations should implement rules for data entry and maintain regular audits to identify and correct any inconsistencies.Without proper attention to these inconsistencies, the reliability of data-driven insights diminishes significantly, ultimately harming business strategies.
Complex Data Structures
In modern organizations, data often exists in complex structures. This complexity can stem from the integration of legacy systems, overlapping databases, or the diversity of data formats. Complex data structures make it challenging for analysts to execute effective mapping because understanding relationships among different datasets can be difficult.
To manage this complexity, analysts should assess the existing data architecture before initiating mapping efforts. Tools that visualize data relationships can also be helpful in understanding complex connections. Recognizing patterns and attributes in the data aids in simplifying the data mapping process, leading to more streamlined analysis and integration.
Undefined Data Standards
Undefined data standards can obstruct the data mapping process significantly. When organizations lack established protocols regarding how data should be formatted, labeled, and categorized, inconsistencies multiply. Undefined standards can result in numerous interpretations of the same data type, leading to confusion and inefficiency in data handling.
Adopting established data standards within an organization is crucial. This may involve using frameworks like the Data Management Body of Knowledge (DMBOK) or International Organization for Standardization (ISO) guidelines. Establishing these standards early on helps in aligning teams and ensuring that mapping efforts are based on common understandings.
In summary, recognizing these common challenges in data mapping is essential for creating effective data management strategies. Ensuring data consistency, simplifying complex structures, and establishing clear standards can significantly elevate the success rates of data mapping initiatives.
Best Practices for Effective Data Mapping
Implementing best practices is vital for effective data mapping. Such practices enhance the accuracy and reliability of the data, leading to better integration and management. Following certain principles assists business analysts in navigating the complexities of data mapping and ensures that projects are completed efficiently.
Thorough Requirement Gathering
The success of data mapping largely depends on the clarity and completeness of requirements. Thorough requirement gathering involves engaging with stakeholders to understand their needs and expectations. Effective communication is crucial during this phase. Analysts should hold interviews, workshops, and surveys to collect data on what is necessary for the mapping.
Understanding the context of the data is also important. Is it transactional data, analytic data, or metadata? Each type serves different purposes, and gathering specific details can ensure the mapping meets the intended outcomes. Where possible, it can also be wise to document any assumptions made during this process.
Prioritizing Data Sources
Not all data sources hold the same weight in a data mapping project. Prioritizing data sources can help in focusing efforts on the most important elements first. Analysts should identify critical data sources that impact business functionality. When determining priority, consider the reliability, relevance, and completeness of each data source.
Once priority is established, it should guide the mapping process. This creates efficiency and reduces complexity in translations from source to target systems. Often, integrating high-priority data sources may yield the greatest return on investment, allowing for improved decision making.
Utilizing Data Mapping Tools
Another essential practice is to leverage data mapping tools. These tools can automate many aspects of the mapping process, reducing manual efforts and potential errors. Popular tools like Talend, Informatica, and Microsoft SQL Server Integration Services can be beneficial.
When selecting a tool, consider aspects like:
- User-friendliness: It should be accessible for the team.
- Feature set: Ensure the tool meets the specific needs of the project, such as transformation capabilities or connectivity to various data sources.
- Integration options: The tool should be able to seamlessly integrate into existing systems.
Utilizing these tools effectively can streamline processes, as they often come with visual interfaces to identify and map data sources efficiently.
Implementing these best practices can significantly enhance the effectiveness of data mapping, leading organizations to achieve more reliable and insightful data integration.
Adhering to these principles assists business analysts in navigating through the challenges of data mapping. It also contributes to achieving the larger goals of improved data quality and better decision-making capabilities.
Data Mapping Tools and Software
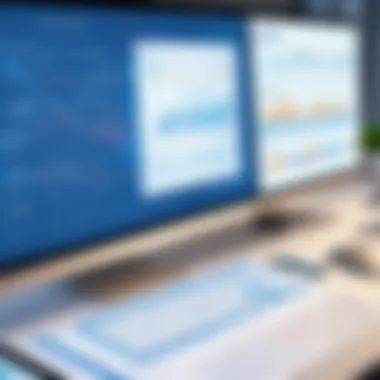
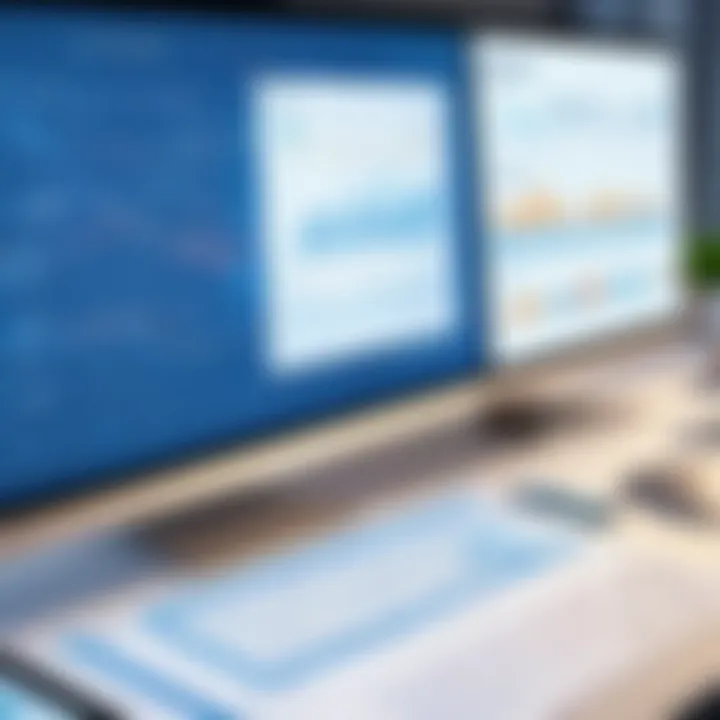
Data mapping tools are critical in the domain of business analysis. They facilitate the process of translating data from one format to another, ensuring that information is actionable and meaningful for various stakeholders in an organization. Recognizing the importance of these tools is essential for maximizing data utility and driving effective data management strategies.
Overview of Popular Tools
Several software solutions exist in the market that are specifically designed for data mapping. Each tool comes with unique features catering to various business needs. Here are some popular tools used in the industry:
- Talend: This open-source integration tool offers robust data mapping capabilities, allowing users to connect diverse data sources and manage complex data transformations intuitively.
- Informatica PowerCenter: A widely-used tool that excels in data integration and has strong mapping functions. It is especially known for its scalability and enterprise-level capabilities, making it suitable for large organizations.
- Microsoft Visio: While primarily a diagramming tool, it can also assist in visualizing data mapping processes effectively, making it easier for teams to collaborate and understand data flows.
- Apache NiFi: Known for its data flow automation, it allows seamless mapping of data between different systems, enhancing operational efficiency.
These tools not only help to streamline the mapping process but also reduce errors and increase the speed of data integration projects.
Comparative Analysis of Features
When choosing a data mapping tool, it is crucial to conduct a comparative analysis of the available features. Here are some key features to consider:
- User Interface: A user-friendly interface is essential to ensure that both technical and non-technical users can navigate the tool effectively. Tools like Talend and Microsoft Visio prioritize usability.
- Integration Capabilities: The ability to integrate with various data sources and formats is vital. Informatica PowerCenter shines in this area, supporting a broad range of connectors.
- Scalability: As businesses grow, their data needs often increase. Tools like Informatica and Talend are designed to scale alongside organizational data demands.
- Cost: Budget constraints are always a consideration. Open-source solutions like Talend can be more cost-effective than enterprise solutions.
- Support and Community: A strong support system and active user community can significantly enhance the user experience. Apache NiFi has an active community that contributes to its ongoing development and troubleshooting.
Choosing the right data mapping tool involves analyzing these features in relation to the specific needs of the organization.
"The right tool enables analysts to focus on delivering actionable insights rather than getting bogged down by technical challenges."
By understanding the different tools and their functionalities, business analysts can make informed decisions that align with their project requirements.
Future Trends in Data Mapping
The landscape of data mapping continues to evolve as technology advances and organizations seek more efficient solutions. Understanding future trends is essential for business analysts, data engineers, and IT professionals. Embracing these trends can lead to improved data management and better decision-making processes.
Automation in Data Mapping
Automation is becoming a fundamental aspect of data mapping. As organizations handle larger volumes of data, manual processes can no longer keep pace. Automation tools can streamline data mapping tasks, reducing the time and effort required. They allow for faster data integration and increase the overall accuracy of data transfers.
- Increased Efficiency: Automated tools can process data mapping tasks quickly, freeing up analysts to focus on higher-level analysis instead of repetitive tasks.
- Error Reduction: Manual mapping is prone to errors. Automated systems minimize these risks, ensuring higher data quality.
- Cost-Effectiveness: Although initial investments in automation might seem high, long-term savings on manual labor and error correction can greatly outweigh these costs.
Impact of Artificial Intelligence
The infusion of artificial intelligence into data mapping processes is another emerging trend. AI technologies can enhance data mapping in various ways:
- Intelligent Data Classification: AI can analyze data sets to categorize and map data structures automatically, improving the speed and accuracy of data mapping processes.
- Predictive Analytics: AI systems can predict potential data integration issues by analyzing historical data mapping projects, allowing teams to proactively address challenges before they arise.
- Natural Language Processing: This allows users to interact with data mapping tools using simple language, reducing the learning curve for analysts.
"AI is transforming how businesses manage and interpret their data. In data mapping, it offers insights and efficiencies previously unimaginable."
Case Studies of Successful Data Mapping Projects
Case studies provide tangible evidence of how data mapping can be effectively utilized in various contexts. These examples highlight the significance of clear, precise mapping strategies tailored to specific organizational needs and can serve as a guide for others in similar domains. Furthermore, they demonstrate the practical benefits that can be reaped from well-executed data mapping projects.
In this section, we will outline several industry-specific examples that showcase successful data mapping initiatives. Each example will offer insight into the methods used and the changes implemented, emphasizing the importance of a tailored approach. The lessons learned will also be discussed to impart knowledge that can be applied to future projects.
Industry-Specific Examples
- Healthcare Sector
A prominent healthcare provider undertook a data mapping project to improve patient data integration across various systems. By defining standardized data fields and implementing a comprehensive mapping strategy, they enhanced their electronic health records. This resulted in more accessible patient information for healthcare professionals, reducing errors and improving patient care outcomes. - Retail Industry
An established retailer faced challenges with inventory management due to inconsistencies between their e-commerce platform and physical stores. Utilizing data mapping, they synchronized product data between these channels. This integration allowed for real-time inventory updates, reducing overstock and stockouts, which directly contributed to increased sales and customer satisfaction. - Financial Services
A financial services company aimed to streamline their reporting processes. They executed a data mapping project that linked disparate data sources, consolidating information into a single reporting tool. As a result, they provided more accurate and timely reports, aiding strategic decision-making and compliance with regulatory requirements.
Lessons Learned
From the highlighted examples, several key lessons emerge that can guide future data mapping endeavors:
- Clarity in Objectives: Every project must start with a clear understanding of what needs mapping and why. Establishing specific goals can prevent scope creep and ensure alignment among stakeholders.
- Involvement of Stakeholders: Engaging users and data owners during the mapping process is crucial. Their insights help to identify relevant data fields and potential issues early in the project.
- Iterative Approach: Mapping should not be a one-time task. An iterative revision based on feedback and changes in data sources is essential for maintaining data quality and relevance.
- Leveraging Technology: Choosing the right data mapping tools is paramount. Platforms like Talend or Informatica can significantly ease the mapping process, automate tasks, and improve accuracy.
"Successful data mapping does more than just connect data; it builds pathways for meaningful insights and improved decision-making."
These insights from real-world applications reiterate that a thoughtful, designed approach significantly impacts the success of data mapping strategies across various industries.