Choosing the Ideal Data Science Learning Platform
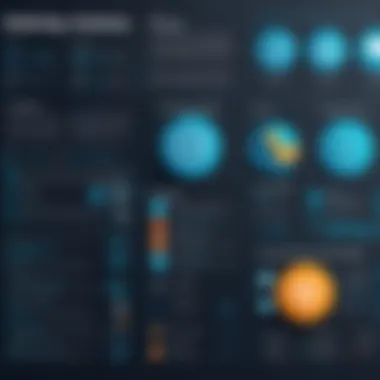
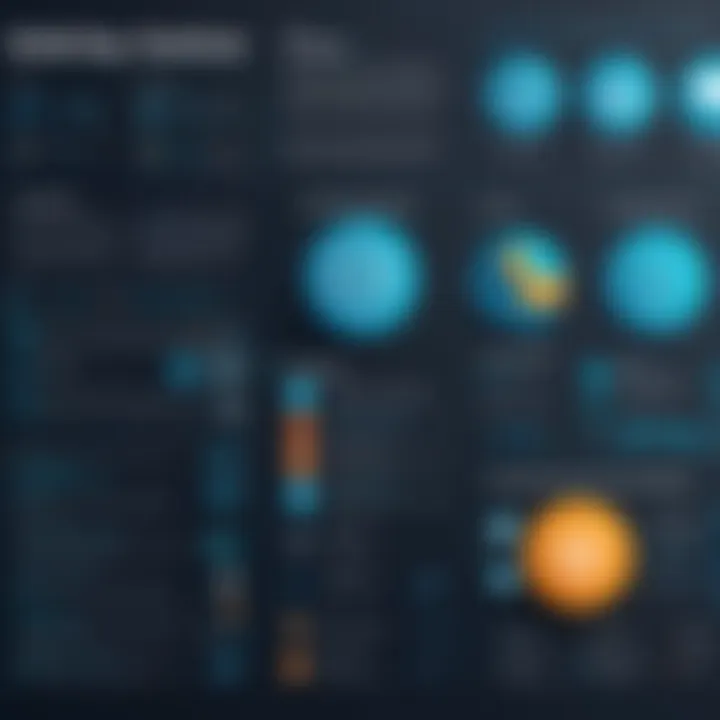
Intro
In today’s rapidly evolving technological landscape, the demand for skilled data scientists is unprecedented. This necessitates a robust and effective learning environment that caters to diverse needs. As professionals and students seek to enhance their knowledge and skills, the choice of learning platform becomes critical. An ideal platform should not only provide quality educational content but also facilitate user engagement and community interaction. The myriad options available can often overwhelm potential learners. Therefore, it is essential to systematically evaluate these platforms to identify which one aligns best with individual learning goals.
This article will delve into the various features of data science learning platforms, aiming to illuminate their capabilities. We will analyze these platforms’ curriculum quality, user interface, and community support. Additionally, we will examine their strengths and weaknesses to provide a comprehensive overview. By the end of this reading, you will be equipped with insights necessary for making an informed decision.
Key Features
Overview of Features
An effective learning platform offers a variety of features that enhance the educational experience. Various aspects should be considered:
- Curriculum Structure: This encompasses the depth and breadth of course offerings, including foundational theories and practical applications.
- Interactivity: Features that promote user participation, such as quizzes, discussion forums, and interactive projects can significantly increase learner engagement.
- Assessment and Feedback: Regular assessments and constructive feedback mechanisms are vital. They help learners gauge their progress and understand areas needing improvement.
- Accessibility: Platforms should provide resources that are easy to access, including accommodating users with disabilities.
Unique Selling Points
Each learning platform typically has unique selling points that distinguish it from its competitors. Here are a few notable examples:
- Coursera: Offers courses from prestigious universities and institutions, ensuring high-quality content.
- Kaggle: Focuses on practical, hands-on projects which can greatly enhance real-world data science skills.
- DataCamp: Provides highly interactive coding environments, promoting learning through doing.
"Choosing the right data science learning platform can significantly impact your career trajectory and knowledge depth."
Performance Evaluation
Speed and Responsiveness
The performance of a learning platform has a direct effect on user experience. Speed and responsiveness should be prioritized when evaluating platforms. A platform that loads quickly and responds promptly to user inputs can reduce frustration and facilitate seamless learning.
Resource Usage
Resource usage is another critical aspect. Platforms should optimize resource consumption to ensure they function smoothly across various devices. Efficient resource management can lead to a more enjoyable learning experience, preventing lagging or crashing, especially during intensive coding sessions or live tutorials.
Intro to Data Science Education
In the digital age, the relevance of data science is undeniable. Organizations across industries increasingly rely on data to make informed decisions. The importance of learning data science cannot be overstated. This section introduces the foundations of data science education. Understanding these foundations prepares learners for a complex field that merges statistics, programming, and domain expertise. The objective is to underscore the necessity of this knowledge in today’s job market.
Defining Data Science
Data science is commonly regarded as a field that combines various disciplines, including statistics, computer science, and domain expertise. It focuses on extracting insights from structured and unstructured data. The role of a data scientist involves analyzing data to find patterns, trends, and correlations. This process requires various tools and methodologies, from data cleaning to statistical modeling. In essence, defining data science sets the stage for understanding its significance and applications.
Importance of Learning Data Science
The growing demand for data science skills among employers highlights the importance of education in this domain. Pursuing data science education opens numerous career opportunities. Professionals equipped with these skills can contribute to decision-making processes and innovation within their organizations. Additionally, learning data science aids in developing critical thinking and problem-solving skills, which are vital in any career path.
"Data education is essential not just for careers but also for informed citizenship in a data-driven world."
For those interested in entering this field, identifying the right learning platform is crucial. The right education can shape a learner’s experience and enhance their proficiency. Understanding data science and its relevance prepares learners for the challenges ahead.
Criteria for Evaluating Learning Platforms
In the increasingly competitive landscape of data science education, selecting the right learning platform can significantly impact the success of learners. The criteria for evaluating these platforms are essential in guiding individuals, especially software developers, IT professionals, and students, towards an informed choice.
When considering online learning options, it is crucial to focus on specific elements that can enhance the training experience and ensure the acquisition of practical skills. The right criteria can assist in understanding which platform aligns with individual learning goals and professional requirements. Below are the core aspects to consider when evaluating learning platforms:
- Curriculum and Content Quality
- Ease of Use and Accessibility
- Instructor Expertise
- Community Engagement and Support
Each of these elements serves as a pillar on which the overall effectiveness of a learning platform stands. They help address the varied needs of users, helping to filter out platforms that do not meet high educational standards.
A careful assessment of these factors not only fosters a streamlined learning experience but also cultivates a deeper understanding of data science concepts. Moreover, it enables learners to maximize their return on investment in education, both financially and in terms of time commitment.
Curriculum and Content Quality
The quality of the curriculum is a critical determinant of a platform's effectiveness. A well-designed curriculum should cover essential data science fundamentals and advanced topics. Learners should expect a combination of theoretical concepts alongside practical applications. Look for platforms offering a mix of instructional videos, interactive exercises, and real-world projects that challenge and enhance comprehension.
Moreover, keep an eye on the relevance and currency of the content. Data science is a rapidly evolving field. Outdated information can hinder a learner's ability to stay competitive. Regular updates and refreshes of course materials are a good sign of a platform's commitment to quality.
Ease of Use and Accessibility
User experience cannot be overlooked. A platform should be intuitively designed, allowing learners to navigate with minimal frustration. Complicated interfaces can hamper learning. Features like multimedia resources, easy navigation, and mobile compatibility greatly enhance the learning experience.
Accessibility is equally important. Platforms need to be usable on various devices and should accommodate learners with different needs. This inclusivity can make a significant difference in user satisfaction and retention rates. A good user interface not only aids engagement but also optimizes the learning process.
Instructor Expertise
Instructors bring knowledge and experience to the educational journey. Evaluating the expertise of instructors is vital. Look for platforms that provide information about their instructors' qualifications and industry experience. Instructors should possess a sound understanding of both theoretical frameworks and practical applications. Engaging instructors can also inspire and motivate learners, which is essential for retaining interest in the subject.
Community Engagement and Support
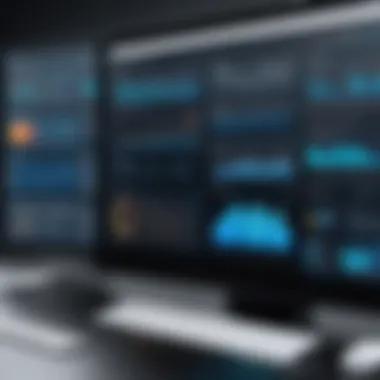
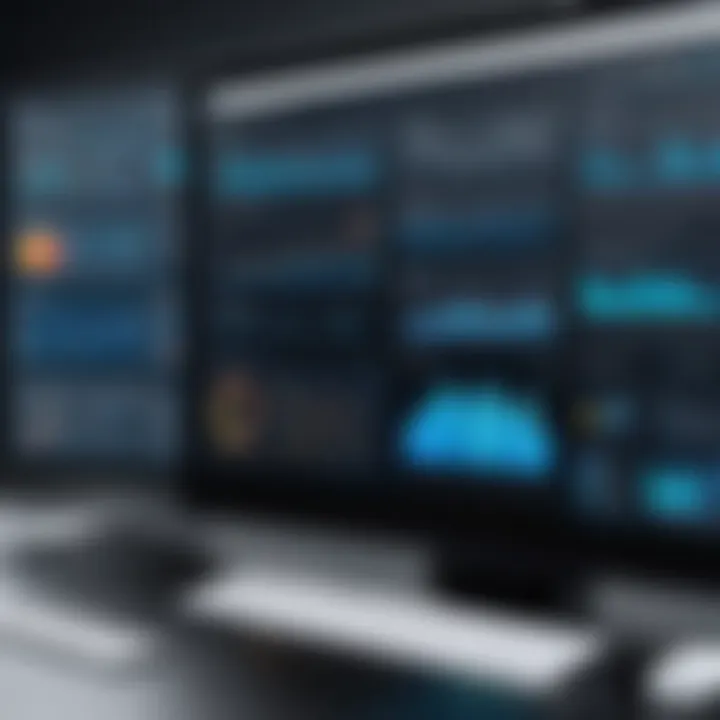
A supportive learning community contributes to the overall experience. Explore platforms that facilitate interaction between learners and instructors, as well as among peers. Learning forums, discussion groups, and direct channels with instructors can enhance understanding and engagement.
Access to support resources, such as mentorship programs, Q&A sessions, and documentation, is crucial. Effective community support can bridge the gap when learners encounter challenges, fostering an encouraging environment that promotes continuous learning.
Top Learning Platforms for Data Science
Selecting the appropriate learning platform for data science is crucial. This can influence your educational trajectory and career prospects. There are numerous platforms available, each with distinct offerings that cater to different learning styles and objectives. Choosing the right one can help streamline the learning process and enhance knowledge retention.
In this section, we will review four notable platforms, highlighting their unique characteristics, curriculum content, user feedback, and both advantages and disadvantages. By assessing these aspects, learners can better understand how each platform aligns with their personal and professional goals.
Platform One: Overview and Features
Curriculum Details
The curriculum of Platform One is comprehensive and provides a well-rounded foundation in data science. The inclusion of practical projects alongside theoretical concepts is a significant characteristic. This approach reinforces understanding and application of skills.
A unique feature of this curriculum is the integration of industry-standard tools, such as Python and R. This adds relevance to the learning experience, preparing students for real-world applications. The trade-off may be the depth of coverage for some niche topics, which can be less extensive.
User Feedback
Feedback from users of Platform One often emphasizes the clarity of the instructional materials. Learners appreciate the structured lessons that lead them step by step through complex topics. However, some users report that the peer interaction could be improved.
The ability to submit assignments and receive timely feedback adds value. This feature enhances the learning journey by allowing users to track progress and understanding.
Pros and Cons
The advantages of Platform One include a robust curriculum and supportive user community. However, it may lack in areas like personalized learning paths which can be a disadvantage for some learners.
Pros:
- Comprehensive content
- Strong community support
- Real project work
Cons:
- Limited niche topic coverage
- Need for improved peer interaction
Platform Two: Overview and Features
Curriculum Details
Platform Two's curriculum stands out due to its modular approach. This allows learners to pick and choose modules based on their interests and career goals. Such customization is appealing but may make the structure less coherent for beginners.
Unique to this platform is its emphasis on data ethics and responsible AI. This is essential as these topics gain prominence in industry discussions. However, beginner students might find some modules challenging without foundational knowledge.
User Feedback
User feedback indicates a high level of satisfaction with the interactivity of the course materials. Users find that the mix of video lectures, quizzes, and interactive exercises helps assimilate difficult concepts.
A disadvantage noted by some users is the occasionally overwhelming volume of material available, which can lead to decision fatigue.
Pros and Cons
The strengths of Platform Two are its flexibility in learning path and emphasis on ethics in data science. However, the disadvantage is that this might not suit every learner’s style, particularly those who prefer a structured approach.
Pros:
- Customizable learning paths
- Focus on ethics in data science
Cons:
- Overwhelming content volume
- Less structured for beginners
Platform Three: Overview and Features
Curriculum Details
Platform Three offers a curriculum that is heavily oriented toward practical applications. Courses are designed with input from industry professionals, ensuring that what is taught is current and relevant to market needs.
One unique aspect is the inclusion of case studies from well-known companies, giving learners insights into real-world data challenges. The downside is that such a focus on application may gloss over theoretical underpinnings which are also vital.
User Feedback
User reviews often underscore the quality of the mentorship provided. Many learners have highlighted the direct support from industry experts as a major motivator during their studies. Nonetheless, some have found certain courses to lack sufficient depth on technical topics.
Pros and Cons
The advantages of Platform Three include its industry relevance and support from experts. The downside could be the potential lack of depth in purely academic discussions.
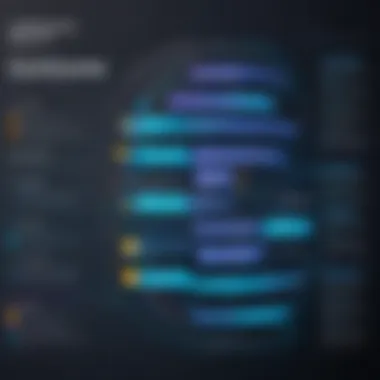
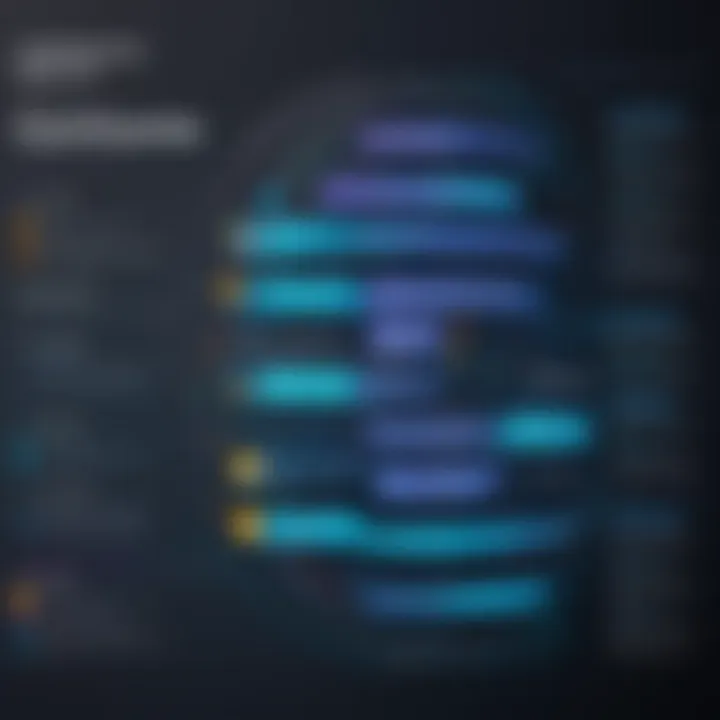
Pros:
- Industry-driven courses
- Excellent mentorship options
Cons:
- Limited theoretical exploration
- Some courses may feel shallow
Platform Four: Overview and Features
Curriculum Details
Platform Four presents a uniquely collaborative learning environment. It offers a variety of courses that focus not only on technical skills but also on teamwork and communication within data science projects. This collaborative emphasis prepares students for a professional environment where teamwork is key.
The curriculum is rich in project-based learning which helps bridge the gap between theory and practice. However, the collaborative model might not suit individuals who prefer independent study.
User Feedback
Feedback on Platform Four often mentions the quality of peer-to-peer learning experiences as a strong positive. Many users value the discussions and group projects, considering them beneficial for skill enhancement. The reliance on group dynamics can sometimes disadvantage those who learn better in solitary conditions.
Pros and Cons
The engaging learning experience of Platform Four is its primary strength, offering strong collaboration opportunities. Contrarily, the cons involve possible challenges for those who thrive in solitary study environments.
Pros:
- Collaborative project work
- Strong community aspect
Cons:
- May not fit independent learners
- Group dynamics variability
Learning platforms vary significantly in their offerings for data science education. Each reviewed platform has distinct advantages and potential drawbacks. Understanding these details will empower learners to select the platform that best meets their specific needs.
Cost Considerations
Understanding the cost of data science learning platforms is crucial for anyone entering this field. The financial commitment varies significantly across different platforms. This section examines pricing structures and return on investment, helping learners determine the value of their choices.
Pricing Models of Learning Platforms
Learning platforms offer a range of pricing models. These vary to meet diverse learner needs and budgets. Here are some common models:
- Subscription-Based: This approach allows users to pay a recurring fee, typically monthly or annually. Platforms such as Coursera and DataCamp use this model, giving access to a vast array of courses.
- Pay-Per-Course: Here, learners pay for individual courses. This model is beneficial for those who want to focus on specific skills without committing to long-term subscriptions. Platforms like Udemy follow this method.
- One-Time Payment: Some platforms offer a comprehensive package for a single fee. This could include access to multiple courses and resources. For example, Codecademy provides such options for in-depth learning.
- Free or Freemium Models: These allow learners to access foundational content free of charge, with options to upgrade for advanced materials. This model is common on platforms like Khan Academy and edX.
Choosing the right model depends on an individual's financial situation and learning goals.
Return on Investment for Learners
Evaluating return on investment (ROI) is essential when selecting a learning platform. Learners aim to gain skills that can enhance their professional prospects. Thus, an initial cost can lead to significant long-term gains. Factors to consider include:
- Career Advancement: A robust data science course can open doors to better job roles or promotions. These outcomes can often outweigh the costs.
- Skill Acquisition: The quality of skills taught can directly affect job performances. Platforms that offer interactive and practical experiences tend to provide better value.
- Networking and Community: Some platforms include community engagement opportunities. Engaging with industry professionals can lead to jobs or collaborations, enhancing overall ROI.
"Investing in education can yield rich dividends, as the most successful professionals continuously learn and adapt."
In summary, potential learners should analyze both the costs and expected benefits carefully. Each dollar spent should ideally translate into enhanced job readiness and marketability.
User Profiles and Learning Paths
Understanding user profiles and learning paths is essential in the context of selecting the best learning platform for data science. Every learner has unique needs based on their background, experience, and goals. By categorizing users into distinct profiles, platforms can offer tailored resources that optimize the learning process. This not only increases efficiency but also enhances motivation and engagement.
When looking for a data science learning platform, it is important to consider how well it aligns with your user profile. Beginners require foundational knowledge and supportive environments. Intermediate learners seek to deepen their skillsets with more complex concepts, while advanced practitioners often desire specialized content to follow industry trends or specific interests. Platforms that respect these variations are more likely to provide a rewarding educational experience.
Beginners: Finding the Right Fit
For beginners, selecting the right learning platform is crucial. At this stage, learners often lack foundational knowledge. They benefit from platforms that offer structured courses focusing on basic principles and practices in data science. Look for programs that introduce core topics like statistics, programming, and data manipulation.
Key aspects for beginners include:
- Clear Curriculum: A well-defined roadmap that logically progresses through topics.
- Interactive Learning: Features such as quizzes, videos, and practical exercises keep learners engaged.
- Community Support: Access to forums or mentorship where beginners can ask questions and seek guidance.
Platforms like Coursera and edX are notable for their beginner-friendly courses, allowing new learners to grasp fundamentals through carefully designed content.
Intermediate Learners: Advancing Skills
Intermediate learners are at a pivotal point in their education. They have grasped basic concepts and now seek to enhance their understanding and apply knowledge effectively. These learners often have specific areas of interest such as machine learning or data visualization.
Ideal platforms for intermediate users provide:
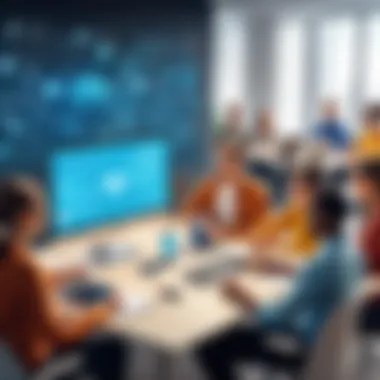
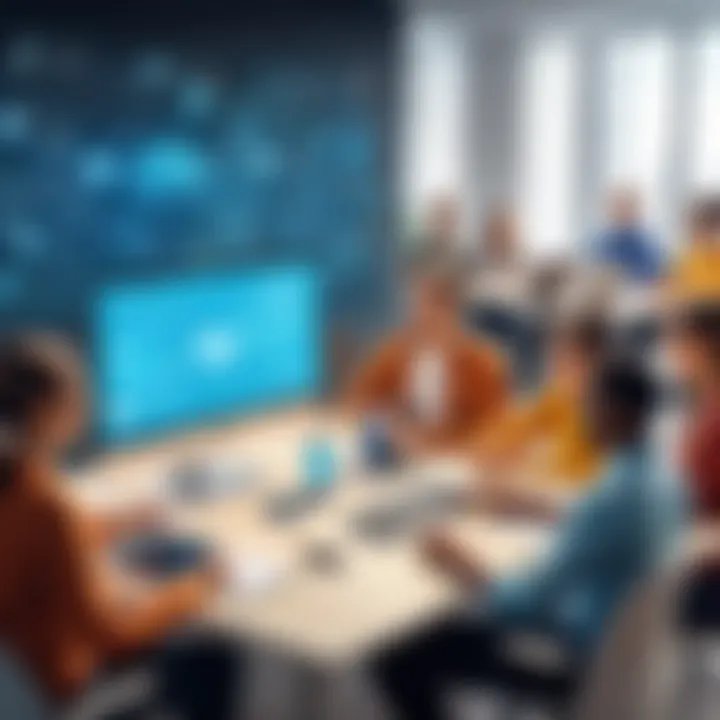
- Advanced Courses: Options that dive deeper into specialized areas.
- Practical Applications: Projects that allow learners to practice and apply concepts.
- Feedback Mechanisms: Tools to assess progress and receive constructive feedback.
Platforms that cater to these needs, such as DataCamp and Udacity, often provide a blend of theory and practice valuable for learners ready to tackle more challenging problems.
Advanced Practitioners: Specialized Learning
Advanced practitioners are often experienced professionals seeking to hone specific skills or explore new frontiers in data science. They usually possess a solid understanding of the fundamental elements and require high-level content that keeps pace with industry advancements.
For this group, the best platforms should offer:
- Specialized Courses: Topics such as deep learning, AI, and ethical considerations in data science.
- Cutting-edge Research: Access to recent studies and innovations within the field.
- Networking Opportunities: Forums for collaboration with other professionals and industry leaders.
Learning options such as Kaggle and LinkedIn Learning often provide relevant resources to meet the sophisticated demands of advanced practitioners, facilitating continued growth and expertise.
Additional Resources and Materials
In the digital age, the quest for knowledge does not end with primary learning platforms. Additional resources and materials play a crucial role in enriching the educational experience in data science. They offer various supportive tools that can enhance comprehension and retention of complex concepts. By utilizing these resources, learners can strengthen their understanding beyond the confines of typical curricula and learning modules.
Supplementary Learning Tools
Supplementary learning tools encompass a wide range of resources, from online quizzes and coding practice platforms to interactive tutorials. These tools can be indispensable for reinforcing theoretical knowledge through practical application. Some notable examples include:
- Kaggle: A platform where users can practice their data science skills by engaging in competitions and projects with real datasets.
- LeetCode: While focused on algorithms and coding interviews, the platform offers problems related to data manipulation that hone programming skills for data-related tasks.
- Codecademy: Provides hands-on coding exercises that cover programming languages essential for data science, like Python and SQL.
These tools not only help in mastering skills but also provide instant feedback, which is crucial for correcting mistakes and understanding complex topics.
Books and Publications in Data Science
For those who prefer traditional learning or require deeper insights, books and publications are invaluable. They often provide a comprehensive look at methodologies and applications in data science. Some recommended titles include:
- "Python for Data Analysis" by Wes McKinney: This book is essential for learning how to use Python for data manipulation and analysis, presented by the creator of Pandas.
- "Deep Learning" by Ian Goodfellow, Yoshua Bengio, and Aaron Courville: A seminal text that covers the fundamental concepts in deep learning and provides insights into its applications.
- "An Introduction to Statistical Learning" by Gareth James et al.: This textbook lays the groundwork for statistical methods used in the analysis and interpretation of data.
Accessing research journals like the Journal of Machine Learning Research, or exploring databases such as ResearchGate can further deepen one’s understanding of current methodologies and innovations in the field.
Additional resources not only supplement learning but often provide real-world applications and examples, enhancing practical skills.
By integrating these supplementary learning tools and literature into one's study regimen, learners can drastically improve their grasp of data science concepts. These resources foster an environment of continuous learning that is vital in the ever-evolving landscape of technology.
Future Trends in Data Science Education
Understanding the future trends in data science education is essential for learners and professionals alike. The field of data science is not static; it evolves rapidly due to technological advancements and shifting market needs. The ability to adapt to these changes is crucial. Embracing continuous education frameworks will help equip individuals with the competencies required to tackle emerging challenges. By focusing on future trends, educators and learners can ensure that the skills they acquire remain relevant and applicable in the job market.
Technological Advancements Impacting Learning
Technological advancements are reshaping the landscape of data science education. Online learning platforms utilize various technologies such as artificial intelligence, machine learning, and interactive tools to enhance the learning experience. For instance, adaptive learning systems analyze students' performance and customize the content accordingly, which helps in targeting specific knowledge gaps.
Some of the prominent trends include:
- Use of Artificial Intelligence: AI-powered tools can facilitate personalized learning paths. By analyzing users’ progress and preferences, these systems can recommend courses or materials suited to individual needs.
- Data Visualization Tools: Applications like Tableau and Power BI provide learners with interactive ways to explore and represent data. Engaging with these tools develops practical skills that are highly sought after in data-driven sectors.
- Virtual Reality (VR) and Augmented Reality (AR): These technologies can create immersive learning environments. For example, a VR simulation could allow students to visualize complex data sets in a three-dimensional space, enhancing their understanding significantly.
Embracing these educational technologies can result in a more enriching learning experience, ultimately leading to better skill acquisition in data science.
Evolving Needs of the Industry
The demands of the data science industry are constantly changing. Companies require professionals who not only possess technical skills but also have the ability to interpret data in a business context. Skills such as data storytelling and ethical considerations in data usage are gaining importance.
Here are some key areas that are evolving:
- Interdisciplinary Knowledge: As businesses integrate data science across departments, having knowledge in areas like finance, marketing, or healthcare will become increasingly vital. Understanding the context in which data is applied can improve decision-making.
- Soft Skills Development: The importance of communication and teamwork is rising. Professionals must articulate complex data insights in a clear manner to stakeholders. Learning environments that foster these soft skills will see greater relevance.
- Focus on Ethics and Compliance: With increasing data regulations, an understanding of ethical implications and compliance will be more important for data professionals. Training that addresses these considerations will prepare learners for contemporary challenges.
Adapting to these evolving industry needs will ensure that educational platforms remain effective and that their graduates are well-prepared for the workforce.
"Continuous education is not just an option; it is a necessity in data science to stay ahead of industry trends."
End
In the realm of data science education, the selection of an effective learning platform holds immense significance. The conclusion of this article not only encapsulates the insights provided but also emphasizes the need for careful consideration of various factors. As explored throughout the sections, each learning platform offers distinct features, teaching methodologies, and community engagement practices. By synthesizing the information from previous sections, readers are better positioned to make informed decisions that align with their individual learning paths and career aspirations.
Choosing the right platform can influence the overall learning experience, from the quality of the curriculum to the level of support available. It is crucial for learners to consider their own goals and prerequisites when evaluating these platforms. The investment in time and resources should yield tangible benefits that enhance understanding and application of data science concepts.
"A well-chosen learning path can accelerate professional growth and open doors to new opportunities in the data science field."
Final Recommendations
After careful examination of the various platforms presented in this article, it's imperative to prioritize specific criteria that resonate most with individual needs. Consider the following recommendations:
- Assess Personal Learning Style: Understand whether you prefer structured courses or self-paced learning. This will dictate your choice significantly.
- Evaluate Curriculum Quality: Ensure that the platform offers a curriculum that covers essential data science topics comprehensively. Look for courses that include hands-on projects and real-world applications.
- Check Community Engagement: A strong community can enhance your learning experience. Platforms that facilitate user interactions and provide ample support should be favored.
- Consider Create Your Learning Path: Identify your career goals and find platforms that offer tailored learning paths suiting beginners, intermediates, or advanced learners.
- Look at Cost vs. Value: Examine the pricing models critically. Ensure that the cost aligns with the value you expect to gain from the platform.
Encouragement for Lifelong Learning
The dynamic nature of data science underscores the importance of lifelong learning. The field is continuously evolving with new techniques, tools, and technologies emerging regularly. Thus, commitment to ongoing education is essential for long-term success. Here are a few encouraging points to consider:
- Stay Updated: Regularly update yourself with the latest trends and advancements in data science methodologies. Attend webinars, join forums like Reddit, and subscribe to relevant publications.
- Network with Professionals: Engaging with peers in the industry can provide insights and inspiration. Participate in meetups or online discussions to share knowledge and experiences.
- Seek New Challenges: Continuously seek projects or courses that push your boundaries. This could be through advanced analytics courses or real-world data projects that stretch your understanding.
- Iterate on Learning: Reflect on what you have learned and adapt your learning strategies accordingly. Keep track of your progress and adjust your focus based on your career trajectory.
Lifelong learning is not just a recommendation, but a necessity in the field of data science. As the industry shifts, remaining agile and responsive to these changes will position you favorably for future opportunities.